Forget cookie-cutter online stores; we're talking about the crème de la crème of e-commerce, where every click feels like it was designed just for you.
Now, if you're still hawking the same generic products to every Tom, Dick, and Harriet who stumbles onto your site, you might as well be using carrier pigeons for your marketing campaigns. In the modern age, personalisation isn't just a luxury—it's a necessity. When it comes to creating personalised shopping experiences, we’re looking at a meticulous orchestration of data analytics, customer behaviour insights, and cutting-edge eCommerce SEO.
So, what's the secret sauce? It's a heady mix of machine learning algorithms, AI-driven recommendations, and a deep dive into SEO services. According to a report by Epsilon, 80% of consumers are more likely to make a purchase when brands offer personalised experiences . That’s a stat worth chasing with a fine-tooth comb.
Dynamic content is the cornerstone here. By leveraging customer data, you can tailor content on-the-fly, ensuring that your homepage doesn't just greet everyone with the same old, tired message. Instead, it welcomes them with open arms, saying, "Hey, we know you, and we've got just what you need." This is where website services come into play, creating dynamic layouts that change based on user data.
Next, let's talk about AI-driven recommendations. You know that feeling when Netflix suggests a show that becomes your new obsession? That’s the magic of machine learning in action. For your e-commerce site, this means recommending products based on past purchases, browsing history, and even what other users with similar profiles have bought. This isn’t just a fancy trick; it’s a proven tactic to boost sales and improve customer satisfaction.
Furthermore, integrating Google Ads services can funnel targeted traffic to your personalised product pages. Google’s machine learning capabilities allow for highly targeted ads that can adapt to user behaviour almost in real-time. Combine this with technical SEO, and you're looking at a potent combination that drives both traffic and conversion rates through the roof.
Of course, personalisation doesn’t stop at product recommendations. The checkout process can be tailored too. Remember the last time you bought something online and the site saved your shipping details, making the checkout process seamless? That’s a result of advanced data integration. Not only does this improve user experience, but it also significantly reduces cart abandonment rates, a bane for many online retailers.
Another aspect to consider is the power of social proof. By showcasing reviews and testimonials from users with similar preferences, you create an environment of trust and relatability. It's like having a personal shopping assistant who knows all your quirks and preferences. This can be further enhanced with Facebook Ads services, which can target lookalike audiences based on your customer data, thus expanding your reach while maintaining a high degree of relevance.
And let's not forget about the visual appeal. High-quality images, videos, and even AR experiences can be personalised to show the most relevant content to each user. For instance, a beauty retailer could use AR to let users virtually try on makeup, which adapts to their skin tone and preferences, creating a highly engaging shopping experience.
Finally, a personalised email marketing strategy can significantly enhance the shopping experience. By sending tailored emails that include product recommendations, exclusive offers, and content relevant to the user’s past interactions with your site, you ensure continuous engagement. This approach can be supercharged with the help of SEO content writing services, ensuring your messages are both engaging and optimised for conversions.
In summary, creating personalised shopping experiences on your website isn't about implementing a single strategy. It's an intricate blend of data analytics, machine learning, dynamic content, and tailored marketing efforts. It's about knowing your customers better than they know themselves and offering them exactly what they want, often before they even know they want it.
By harnessing these strategies, not only do you enhance the customer experience, but you also drive loyalty and, ultimately, sales. It's a win-win situation where everyone walks away happy—except maybe your competitors who are still trying to figure out why their cookie-cutter approach isn't cutting it anymore.
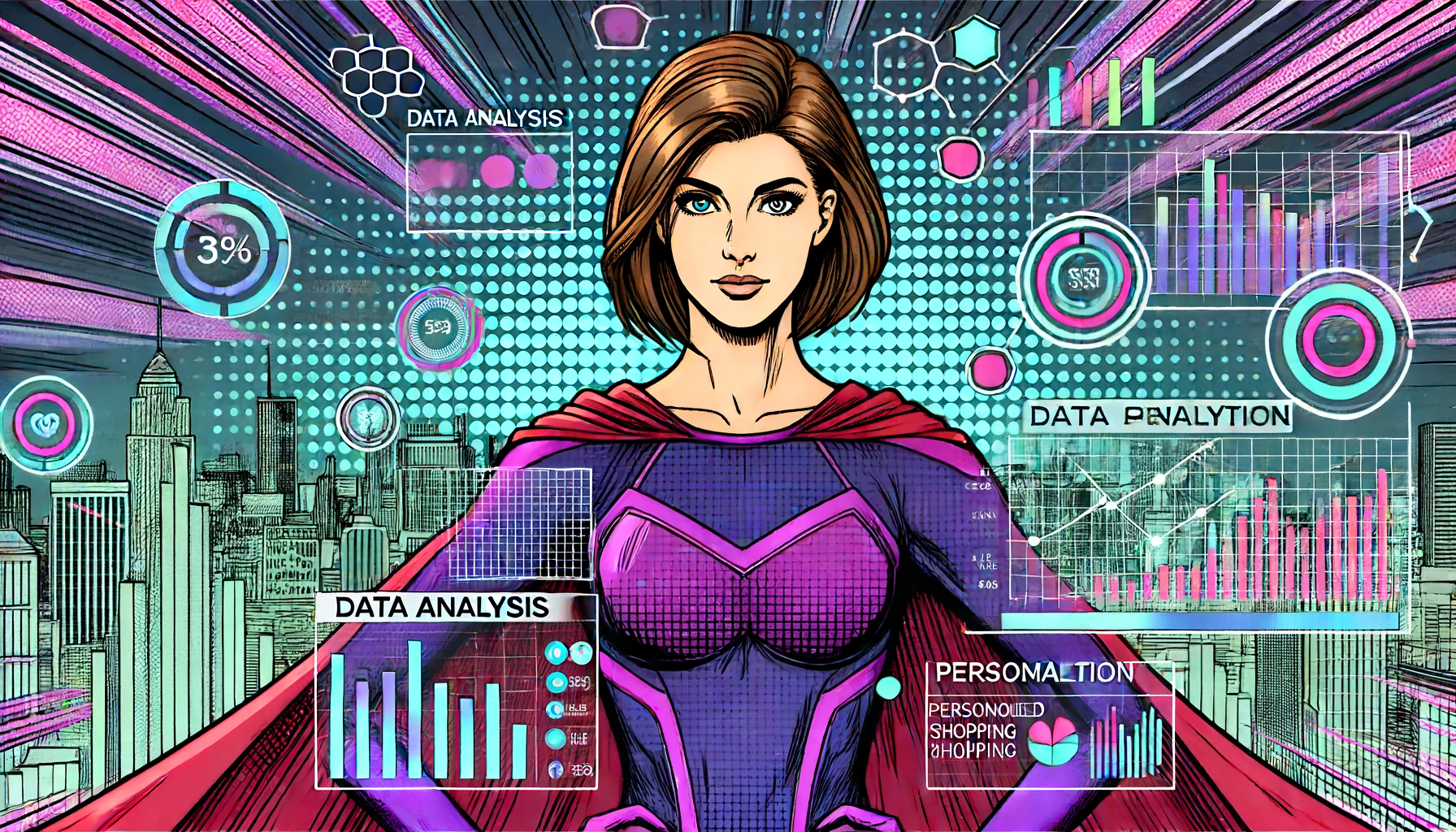
Understanding User Data to Drive Personalisation
If you thought user data was just about tracking a few clicks here and there, you’re in for a revelation. It's more like decoding the human genome but for online behaviour—complex, fascinating, and incredibly powerful.
To kick things off, let’s talk about the types of data we're dealing with. We’ve got first-party data, the treasure trove collected directly from your customers. This includes everything from purchase history and browsing patterns to email interactions and social media engagements. First-party data is the gold standard for personalisation because it's unique to your brand and directly relevant to your customers. Collecting and utilising this data effectively can be facilitated by SEO link building services, ensuring your content reaches the right audience and gathers insightful metrics.
Then there's second-party data, which is essentially someone else’s first-party data shared with you. Partnering with reputable companies can provide valuable insights into your shared customer base, enhancing your personalisation efforts. Last but not least, we have third-party data, collected from various sources and aggregated to provide broader insights into user behaviour. While less precise, it's useful for filling in the gaps and creating a more comprehensive user profile.
Now, once you've gathered all this data, the real fun begins with analysis and segmentation. Segmentation is like crafting a mixtape for your high school crush, but with a lot more data crunching and fewer awkward silences. By segmenting your users based on behaviour, demographics, and preferences, you can tailor your marketing efforts to hit all the right notes. For instance, regular users of enterprise SEO services can be segmented to receive content that dives deep into complex SEO strategies, enhancing their engagement and satisfaction.
Machine learning algorithms play a crucial role here. They sift through mountains of data faster than a caffeine-fueled intern, identifying patterns and predicting future behaviour. This is where predictive analytics comes into play, allowing you to anticipate what products a customer might be interested in before they even realise it themselves. Think of it as your very own crystal ball, but powered by algorithms instead of mysticism.
One of the crown jewels of personalisation is behavioural targeting. By tracking user interactions across your site, you can serve dynamic content that adapts in real-time. For example, a customer lingering on a page for technical SEO services might see a pop-up offering a free consultation or a discount on their first service. This level of responsiveness not only improves user experience but also significantly boosts conversion rates.
Data integration is another vital aspect. Ensuring that all your data sources communicate seamlessly can be a logistical nightmare, but it's essential for a unified personalisation strategy. Modern CRM systems and CDPs (Customer Data Platforms) can consolidate data from multiple touchpoints, providing a 360-degree view of each customer. This integration allows for more sophisticated personalisation tactics, such as synchronising online and offline data to offer a cohesive shopping experience.
Privacy and data security cannot be overlooked. With GDPR and other data protection regulations, ensuring that user data is handled with the utmost care is non-negotiable. Transparent privacy policies and robust data protection measures are critical not only for legal compliance but also for building trust with your customers. Customers are more likely to share their data if they feel it’s being handled responsibly.
Then there’s the power of real-time personalisation. Gone are the days of batch processing data and pushing updates every few weeks. Today, it’s all about real-time interactions. With advanced data processing capabilities, you can adapt your website’s content, offers, and recommendations on the fly, providing a seamless and highly relevant shopping experience. This is particularly effective in high-stakes environments like local SEO services, where immediate relevance can make or break a sale.
To wrap it up, understanding user data isn’t just about collecting and analysing information. It’s about creating a dialogue with your customers, understanding their needs and preferences, and responding with personalised experiences that make them feel valued and understood. By leveraging advanced analytics, machine learning, and real-time data integration, you can transform your e-commerce site into a dynamic, responsive, and highly personalised shopping haven.
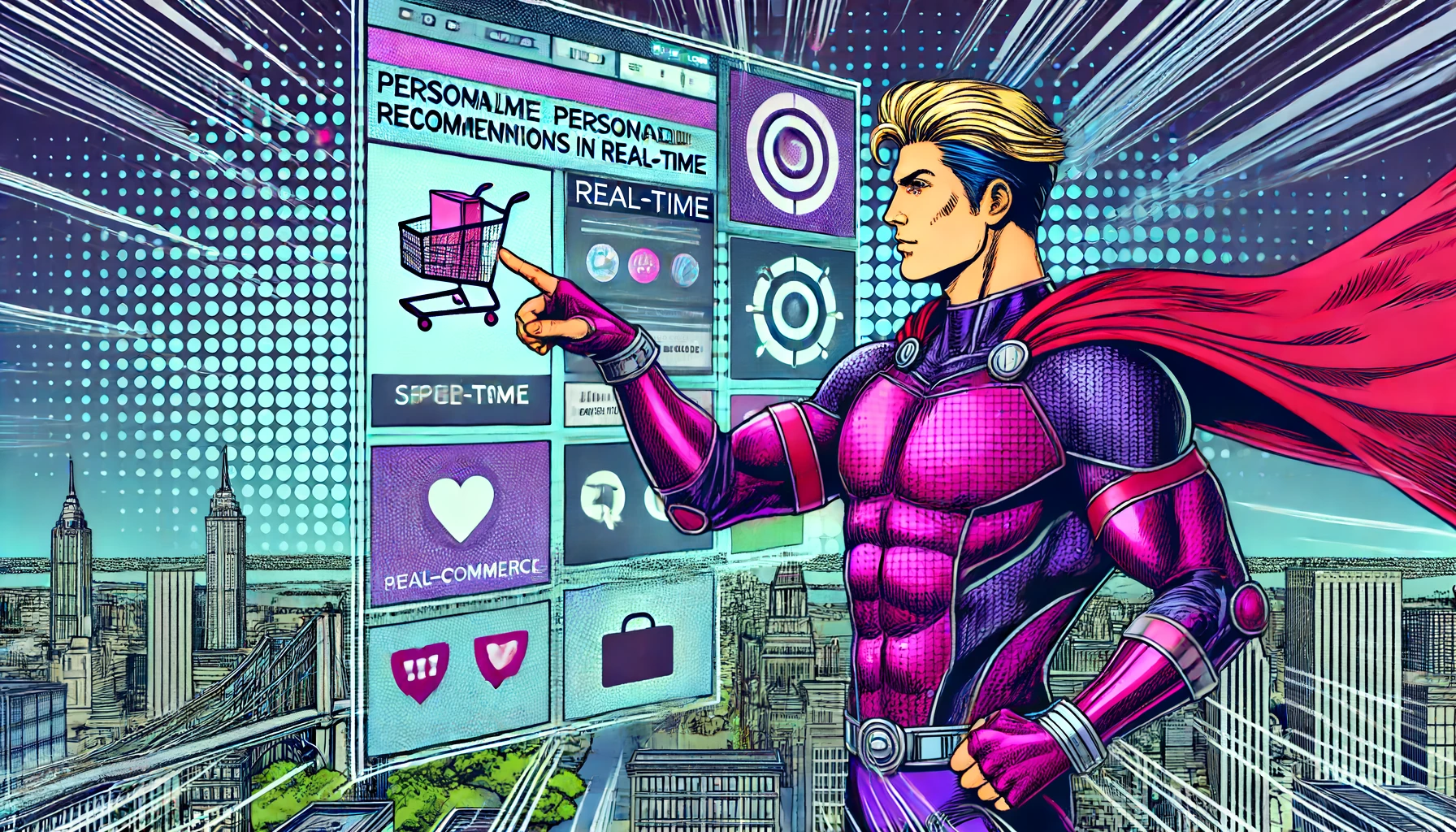
Implementing Real-Time Personalisation on E-commerce Platforms
Ever wondered how your favourite online store seems to read your mind, suggesting the perfect pair of shoes just when your old ones are on their last legs? That’s real-time personalisation at work, my friend, and it's the secret sauce that turns casual browsers into loyal customers.
Real-time personalisation hinges on the immediate processing of user data to deliver tailored content, recommendations, and offers. Unlike static personalisation, which relies on pre-set rules and delayed data processing, real-time personalisation responds instantly to user actions. This dynamic approach requires a robust combination of machine learning algorithms, data processing power, and sophisticated website services.
First off, let's tackle the core of real-time personalisation: data collection. This isn’t just about hoarding user data like a dragon guarding its gold; it’s about collecting the right data at the right time. Clickstream data, for instance, captures every click, hover, and scroll a user makes. Combined with transactional data, social media activity, and historical behaviour, it forms a comprehensive user profile. This profile is the cornerstone of personalised user experiences.
But what happens when this data is collected? Enter real-time data processing. Advanced platforms use in-memory data grids (IMDGs) to process large volumes of data with low latency, ensuring that personalisation actions occur in milliseconds. This is akin to having a hyper-efficient butler who anticipates your every need before you even realise you have one. For instance, an e-commerce site offering enterprise SEO services can instantly adjust its homepage to showcase relevant case studies, articles, or special offers to a returning SEO specialist.
Machine learning models play a pivotal role here. By analysing user data in real-time, these models can predict what products a user is likely to be interested in based on their current and past behaviour. Think of it as your very own recommendation engine, much like the one Netflix uses to keep you binge-watching. These models can be fine-tuned with continuous learning, improving their accuracy over time. This is especially crucial for services like Google Ads, where user preferences and behaviours can shift rapidly.
Personalisation also extends to content. Dynamic content delivery systems ensure that every piece of content a user sees is tailored to their interests and behaviour. For example, a user who frequently searches for SEO content writing services might see a personalised blog post on advanced SEO strategies, while another user interested in technical SEO gets a detailed guide on site audits. This level of customisation enhances user engagement and retention.
Next, we have behavioural triggers, which are essentially real-time responses to user actions. If a user adds a product to their cart but doesn’t proceed to checkout, a personalised pop-up or email can be triggered, offering a discount or highlighting limited stock to create a sense of urgency. These triggers are powered by real-time data and can significantly reduce cart abandonment rates.
Moreover, personalisation isn’t limited to product recommendations and content. It encompasses the entire user journey. Real-time personalisation can adjust the user interface, such as displaying different homepage layouts, altering navigation menus, and highlighting specific features or promotions based on user preferences and behaviour. For instance, a customer looking for local SEO services might see a prominently featured section on local success stories and client testimonials, enhancing their confidence and likelihood of conversion.
Implementing real-time personalisation also involves integrating various systems seamlessly. A robust Customer Data Platform (CDP) can unify data from multiple sources—web, mobile, email, and in-store—providing a holistic view of the customer. This unified data is then used to drive consistent and personalised experiences across all touchpoints. It’s like having an omniscient guide who knows your preferences whether you’re shopping online or visiting a physical store.
Privacy and user consent are critical in real-time personalisation. With regulations like GDPR, ensuring that user data is collected, processed, and stored responsibly is paramount. Users should be informed about data collection practices and given the option to control their data preferences. This transparency builds trust and encourages users to share their data, knowing it will enhance their shopping experience without compromising their privacy.
In conclusion, implementing real-time personalisation on e-commerce platforms isn’t just about fancy algorithms and data crunching. It’s about creating a seamless, intuitive, and highly relevant shopping experience that makes users feel understood and valued. By leveraging advanced data processing, machine learning, and dynamic content delivery, e-commerce platforms can transform casual visitors into engaged, loyal customers, driving growth and competitive advantage.
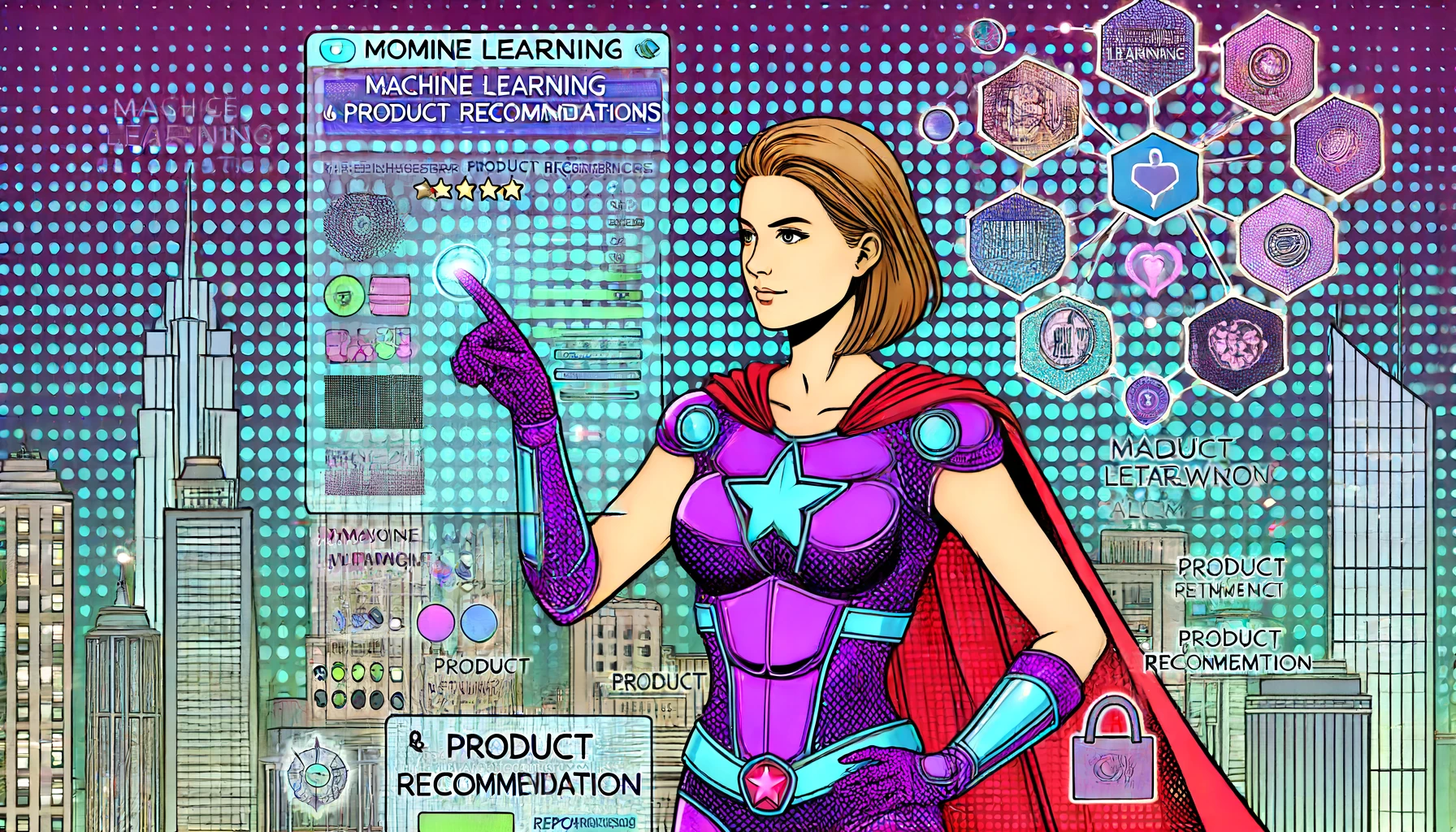
Enhancing Product Recommendations with Machine Learning
Let's talk about the magic behind those eerily accurate product recommendations that make you wonder if your favourite online store has hired a psychic. Spoiler alert: it’s not clairvoyance—it’s machine learning. Buckle up as we dissect how machine learning algorithms elevate product recommendations from mere suggestions to personalised shopping companions.
Machine learning, the brainchild of advanced computer science, thrives on patterns. By analysing historical data and learning from it, machine learning models predict future behaviours with impressive accuracy. In the realm of e-commerce, this translates to recommending products that align perfectly with a user's tastes and preferences. Imagine the precision of a Swiss watch, but for shopping recommendations.
The journey begins with data—lots of it. Every click, view, purchase, and even time spent on a page is valuable. This data feeds into machine learning models, which use techniques like collaborative filtering, content-based filtering, and hybrid methods to churn out recommendations. Collaborative filtering, for example, identifies patterns among users with similar behaviours. If Jane and John both bought the same running shoes, and Jane also bought a fitness tracker, chances are high that John might fancy one too. This method is particularly effective when combined with SEO services, ensuring that the recommendations are visible to users who actively search for related products.
Content-based filtering, on the other hand, focuses on the attributes of items. It suggests products based on similarities to what a user has previously liked or purchased. For instance, if a customer buys a book on advanced SEO techniques, the algorithm might recommend other books on SEO, digital marketing, or analytics. This type of filtering benefits greatly from detailed product descriptions and metadata, which are often enhanced through SEO content writing services.
Hybrid models combine the strengths of both collaborative and content-based filtering, offering a more comprehensive recommendation system. These models can also integrate additional data points such as demographic information, browsing context, and even external factors like seasonal trends. The result is a robust recommendation engine that adapts to various user preferences and behaviours, making it indispensable for complex e-commerce ecosystems.
One of the cutting-edge techniques in machine learning for recommendations is the use of deep learning models. These models, with their intricate neural networks, can capture complex patterns and relationships within data. For instance, a deep learning model can analyse not only what products users bought but also the sequence of their interactions, providing insights into their buying journey. This depth of analysis is akin to having a personalised shopping assistant who understands your tastes better than you do.
Real-time processing is another game-changer. Traditional recommendation systems might update periodically, but real-time processing ensures that recommendations adapt instantly as users interact with the site. This is particularly useful for services like Google Ads, where ad content can be dynamically adjusted based on current user behaviour, maximising relevance and engagement.
Let's not forget the power of A/B testing in refining these models. By continuously testing different recommendation strategies and measuring their impact on user behaviour, businesses can fine-tune their algorithms to achieve optimal results. This iterative process ensures that the recommendation engine evolves alongside user preferences, maintaining its effectiveness over time.
Furthermore, integrating machine learning with customer segmentation enhances personalisation. By segmenting users into distinct groups based on their behaviour and preferences, machine learning models can tailor recommendations more precisely. For example, a frequent buyer of local SEO services might receive recommendations for related localised marketing tools, while a user interested in technical SEO could be shown advanced analytical tools and resources.
Another exciting development is the use of reinforcement learning, where algorithms learn from user feedback in real-time. If a user ignores a recommendation, the system adjusts its model to avoid similar suggestions in the future. Conversely, if a user engages positively with a recommendation, the system reinforces this behaviour, making similar suggestions more often. This dynamic learning process ensures that the recommendation engine remains agile and responsive to user needs.
Finally, transparency and trust are crucial. Users are becoming increasingly aware of how their data is used. Providing clear information about how recommendations are generated and offering control over personal data can enhance user trust and willingness to engage with personalised recommendations. Ensuring compliance with data protection regulations and ethical use of machine learning models is paramount.
In conclusion, enhancing product recommendations with machine learning is about creating a highly personalised, responsive, and engaging shopping experience. By leveraging sophisticated algorithms, real-time data processing, and continuous optimisation, e-commerce platforms can transform their recommendation systems from simple suggestive tools into powerful engines of user satisfaction and sales growth. So next time you get that perfect product suggestion, tip your hat to the marvels of machine learning.

Personalising User Interfaces Based on Behavioural Data
Alright, let’s delve into the world of user interfaces that morph and adapt as if they’ve been sprinkled with a touch of digital sorcery. But there’s no magic here—just the potent power of behavioural data. Personalising user interfaces (UIs) based on behavioural data is like crafting a bespoke suit for each visitor to your website, ensuring a perfect fit that enhances the overall experience and drives engagement.
To start, let's discuss the treasure trove of behavioural data. This includes clickstream data, which captures every interaction a user has with your site—clicks, scrolls, page views, and more. Combining this with data from previous purchases, time spent on specific pages, and even social media interactions provides a comprehensive view of user behaviour. By analysing this data, you can tailor the UI to meet individual user preferences and habits, creating a more intuitive and engaging experience. Think of it as giving each user a personalised tour guide through your digital storefront.
One of the first steps in personalising UIs is segmenting users based on their behaviour. Advanced segmentation techniques can group users by their interaction patterns, purchase history, and even predictive models of future behaviour. For example, a user frequently browsing SEO content writing services might be presented with a customised interface highlighting the latest SEO blog posts, tips, and case studies. This level of segmentation ensures that each user feels the interface is designed specifically for their needs.
Dynamic content is key to personalising user interfaces. By leveraging content management systems (CMS) that support dynamic content, you can change elements of your site in real-time based on user behaviour. This might involve altering banners, product recommendations, and even the layout of the site. For example, a returning user who has previously shown interest in eCommerce SEO could see a homepage that prominently features related services and success stories. This adaptability makes the user feel recognised and valued.
Moreover, machine learning algorithms can predict user needs and adjust the interface accordingly. By analysing patterns in user data, these algorithms can anticipate what a user might be looking for and present it proactively. For instance, if a user often visits the local SEO services section, the site might highlight local SEO tips, case studies, and tools right on the homepage. This predictive personalisation keeps the user engaged by showing them the most relevant content without them having to search for it.
Behavioural triggers can further enhance personalisation. These triggers respond to specific user actions in real-time. For instance, if a user hovers over a particular service, a small popup could appear with more information or a special offer related to that service. This immediate feedback loop makes the interface feel alive and responsive, increasing the likelihood of user interaction and conversion.
Personalisation isn’t limited to just content; it extends to the entire layout and design of the interface. Adaptive layouts can change the positioning of elements based on user preferences and behaviour. For example, a user who frequently accesses certain features or tools could have those elements moved to more prominent positions for easier access. This not only improves usability but also enhances the overall user experience by making the interface more intuitive and user-friendly.
Real-time data processing plays a crucial role here. By using technologies like in-memory data grids and real-time analytics platforms, you can ensure that the UI adapts instantly to user interactions. This means no more waiting for batch processes or delayed updates—the interface responds as quickly as the user interacts with it. For instance, if a user frequently visits the Google Ads service page, the next time they visit, the site can dynamically adjust to feature Google Ads prominently, perhaps with the latest updates or special promotions.
Integrating AI-driven chatbots can also personalise the user interface. These chatbots can provide personalised assistance based on user data, guiding users through their journey with tailored recommendations and support. For example, a chatbot could offer help navigating through the technical SEO services, answering specific queries, or even suggesting additional resources based on the user's browsing history.
Privacy and user consent are paramount in personalising UIs based on behavioural data. Users should always be informed about how their data is being used and have the option to control their data preferences. Transparent privacy policies and easy-to-use data control options build trust and encourage users to engage more deeply with personalised features.
To sum up, personalising user interfaces based on behavioural data is about creating a seamless, intuitive, and engaging experience tailored to each user’s unique preferences and behaviours. By leveraging advanced data analytics, machine learning, and dynamic content systems, e-commerce platforms can transform static interfaces into adaptive, personalised environments that delight users and drive engagement. The future of e-commerce is personal, and those who harness the power of behavioural data will lead the way.
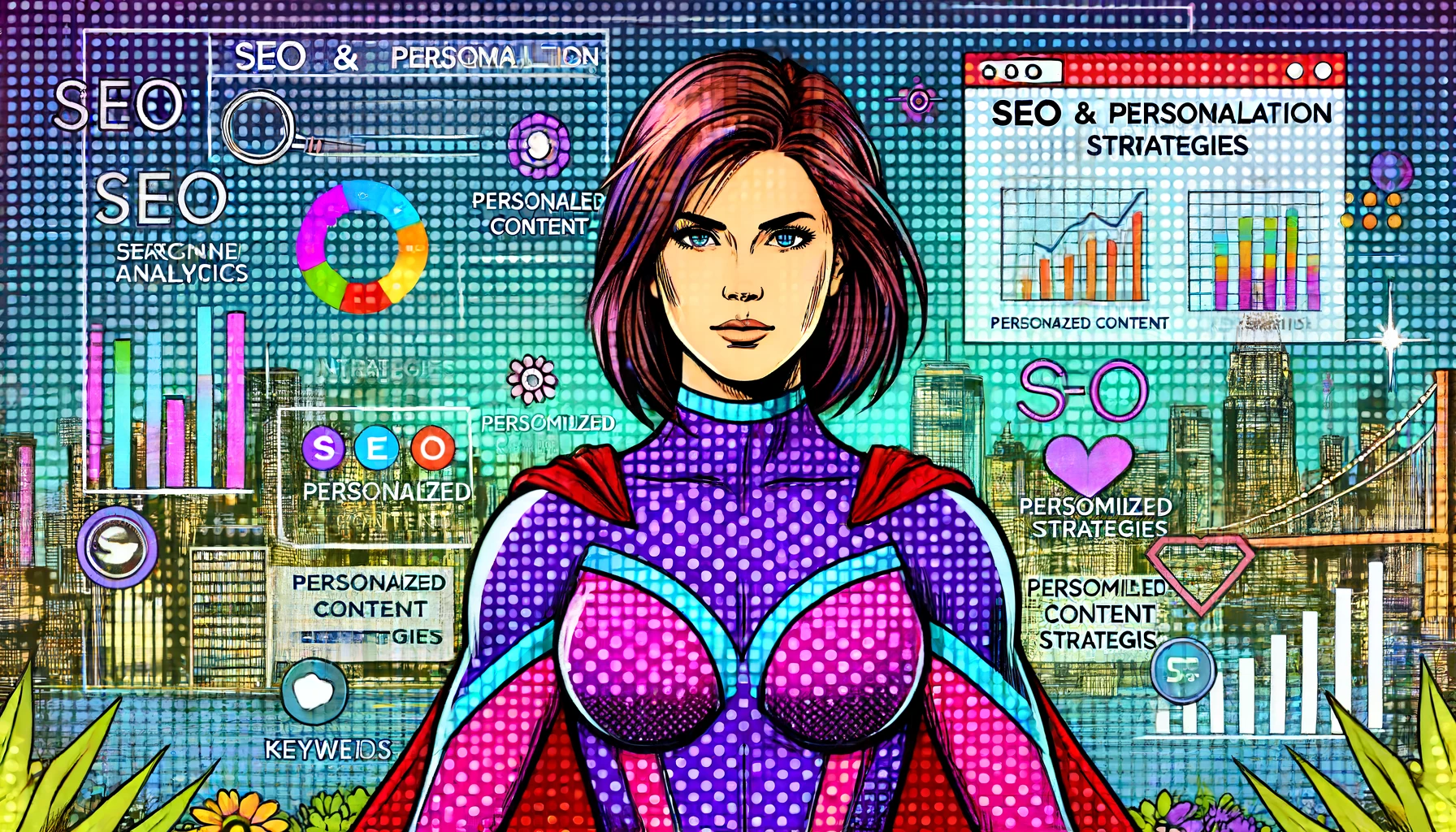
Integrating Personalisation with SEO Strategies
Personalisation and SEO might seem like two parallel paths, but they intersect more than you might think. Personalisation aims to create a unique experience for each user, while SEO focuses on making your site visible to search engines and, by extension, users. When these two strategies align, the result is a highly engaging and discoverable website.
To begin with, let’s talk about the synergy between personalised content and SEO. Personalised content is crafted to meet the specific needs and preferences of individual users. However, to ensure that this content also ranks well in search engines, it must be optimised for relevant keywords and adhere to SEO best practices. For instance, a user interested in eCommerce SEO should be served content that not only speaks directly to their needs but also includes strategically placed keywords that improve search engine rankings.
Dynamic content plays a crucial role here. By leveraging dynamic content frameworks, you can create pages that adapt based on user behaviour and preferences, while still maintaining SEO-friendly structures. For example, a landing page that highlights SEO services can dynamically adjust to show more detailed insights, case studies, or service offerings based on a user’s previous interactions. The key is to ensure that these dynamically generated elements are also crawled and indexed by search engines. Implementing proper schema markup and ensuring that dynamic content is accessible to search engine bots can help achieve this balance.
Another critical aspect is user intent, a cornerstone of both personalisation and SEO. Understanding what users are looking for and delivering content that meets these needs is essential. By analysing search queries and user behaviour, you can tailor your content to address specific intents. For instance, users searching for local SEO services might have different needs compared to those looking for enterprise SEO services. Personalising the content to align with these intents while optimising for the right keywords can significantly enhance both user experience and search rankings.
Let’s not overlook the importance of SEO content writing. High-quality, personalised content that is also SEO-optimised is a game-changer. This involves creating content that is engaging, informative, and tailored to the user’s journey while ensuring it is keyword-rich, well-structured, and includes internal and external links. For example, a blog post tailored for users interested in technical SEO can be crafted to provide deep insights and actionable tips, while also linking to relevant internal pages and authoritative external sources.
Moreover, machine learning algorithms can enhance both personalisation and SEO. These algorithms analyse vast amounts of data to predict user behaviour and preferences, allowing for more precise personalisation. Simultaneously, they can identify emerging trends and keywords, helping to adjust SEO strategies in real-time. For instance, if a significant number of users start showing interest in a new SEO technique, the machine learning model can highlight this trend, prompting the creation of targeted content that is both personalised and SEO-optimised.
Another innovative approach is leveraging A/B testing for SEO and personalisation. By testing different versions of content, layouts, and calls-to-action, you can determine what resonates best with users while also observing which variations perform better in search rankings. This iterative process ensures that your personalised content is not only engaging but also optimised for search engines.
Furthermore, integrating personalisation with local SEO strategies can drive significant results, especially for businesses targeting specific geographic areas. By personalising content based on the user’s location and search behaviour, you can create highly relevant experiences that improve local search rankings. For instance, a user searching for local SEO tips in Sydney could be presented with content that includes local case studies, success stories, and tailored service offerings, enhancing both engagement and local search visibility.
Personalisation also extends to technical SEO. Ensuring that personalised elements of your site load quickly, are mobile-friendly, and are easily navigable is crucial. Techniques such as lazy loading for images, optimising JavaScript for dynamic content, and ensuring a responsive design are vital. These technical aspects not only enhance user experience but also play a significant role in improving search engine rankings.
Lastly, it’s essential to maintain a balance between personalisation and privacy. With regulations like GDPR, ensuring that user data is handled responsibly is paramount. Transparent data practices and offering users control over their data not only build trust but also encourage users to share information that enhances personalisation. This trust, in turn, leads to more effective personalised experiences and better SEO outcomes, as search engines favour sites that are both user-friendly and compliant with data regulations.
In summary, integrating personalisation with SEO strategies is about creating a seamless blend of tailored user experiences and search engine-friendly content. By leveraging dynamic content, understanding user intent, and employing advanced machine learning algorithms, businesses can enhance both personalisation and SEO performance. The result is a website that not only ranks high on search engines but also delivers highly engaging and relevant experiences to every visitor.
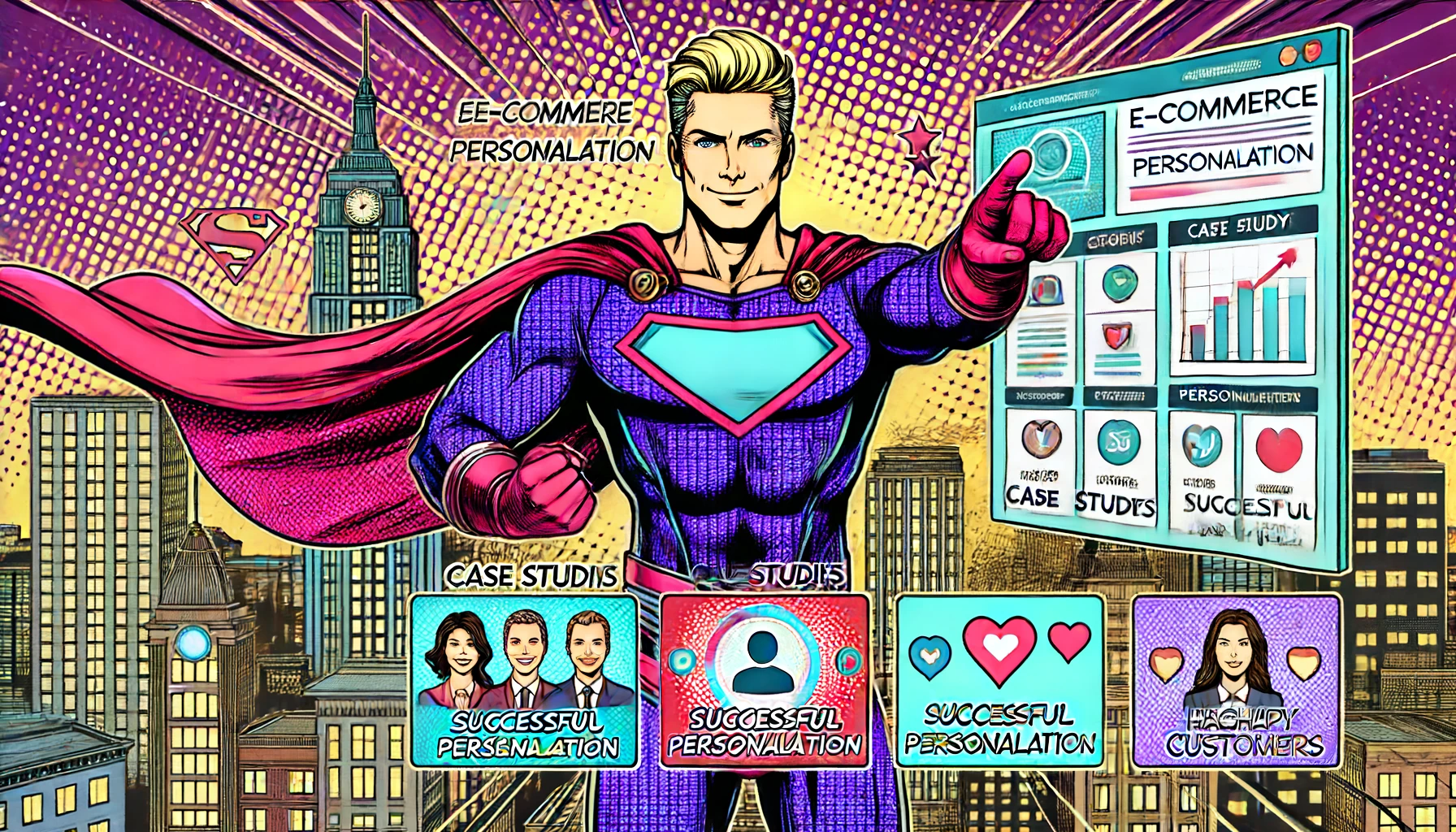
Case Studies: Successful Personalisation in E-commerce Websites
Let’s dive into the rich, detail-laden world of personalisation in e-commerce, where brands have leveraged cutting-edge strategies to create highly personalised shopping experiences that not only delight customers but also significantly boost conversions and loyalty. Here, we’ll explore some of the most successful case studies that highlight the power of personalisation.
1. Amazon: The Pinnacle of Personalised Recommendations
Amazon’s success story in personalisation is legendary. The retail giant utilises a complex recommendation engine powered by machine learning algorithms to deliver highly personalised shopping experiences. By analysing user behaviour, purchase history, and even browsing patterns, Amazon’s system predicts what products a user might be interested in and displays these suggestions prominently on their homepage and throughout their browsing journey.
Key Strategy: Collaborative Filtering and AI-Driven RecommendationsResults: Approximately 35% of Amazon’s sales come from its recommendation engine, highlighting the immense impact of personalised suggestions.
By continuously refining its algorithms, Amazon ensures that its recommendations remain relevant and timely, driving both customer satisfaction and sales. The integration of these personalised recommendations with robust eCommerce SEO ensures that the products are also optimised for visibility, increasing the likelihood of discovery through organic search.
2. Netflix: Tailoring Content to Viewer Preferences
Netflix is another prime example of how personalisation can transform user experience. By leveraging data analytics and machine learning, Netflix provides personalised content recommendations that keep users engaged and coming back for more. The platform analyses viewing habits, search history, and even the time of day users watch content to suggest movies and shows that match their preferences.
Key Strategy: Machine Learning and Predictive AnalyticsResults: Over 80% of the content watched on Netflix is based on personalised recommendations.
Netflix’s ability to personalise content extends to creating custom thumbnails for shows and movies, tailored to appeal to individual users. This deep level of personalisation not only enhances user engagement but also optimises content discovery, making it a powerful tool in retaining subscribers.
3. Spotify: Personalised Playlists and Discover Weekly
Spotify’s personalisation strategy revolves around creating unique, tailored music experiences for each user. The platform’s famous “Discover Weekly” playlist uses collaborative filtering, natural language processing, and audio analysis to recommend new songs based on a user’s listening history and preferences.
Key Strategy: Collaborative Filtering and Audio AnalysisResults: “Discover Weekly” has become a cornerstone of user engagement, with millions of users streaming the personalised playlists every week.
Spotify’s approach ensures that users are constantly discovering new music that aligns with their tastes, significantly enhancing user satisfaction and retention. By integrating these personalised experiences with SEO strategies such as optimising artist and track metadata, Spotify ensures that users can easily find and engage with their favourite music.
4. Stitch Fix: Personalised Fashion Recommendations
Stitch Fix combines data science with human insight to deliver personalised fashion recommendations to its customers. Users fill out a detailed style profile, and Stitch Fix’s algorithms, along with human stylists, curate a selection of clothing items tailored to individual tastes and preferences.
Key Strategy: Hybrid Approach Combining Data Science and Human ExpertiseResults: Stitch Fix’s personalised styling service has attracted over 3 million customers, showcasing the power of tailored recommendations.
This hybrid approach not only enhances the accuracy of recommendations but also provides a human touch that many customers appreciate. Stitch Fix’s model demonstrates the effectiveness of combining advanced algorithms with human creativity to deliver personalised shopping experiences.
5. Sephora: Personalised Beauty Solutions
Sephora leverages a comprehensive personalisation strategy to enhance the shopping experience both online and in-store. Through the use of AI and AR technologies, Sephora provides personalised product recommendations, virtual try-ons, and tailored beauty advice.
Key Strategy: AI and AR Technologies for Virtual Try-Ons and Personalised RecommendationsResults: Sephora’s digital tools, such as the Virtual Artist, have significantly boosted user engagement and conversion rates.
By integrating AI-driven personalisation with AR experiences, Sephora allows users to virtually try on products and receive personalised beauty tips, creating a highly engaging and interactive shopping journey. This strategy not only enhances customer satisfaction but also drives higher sales conversions.
6. Nike: Personalised Shopping with NikePlus
Nike’s NikePlus membership program offers a personalised shopping experience that includes tailored product recommendations, exclusive access to new releases, and customised workout plans. The program leverages data from users’ interactions with the Nike app, website, and physical stores to create a seamless and integrated experience.
Key Strategy: Integrated Omnichannel PersonalisationResults: NikePlus has significantly increased customer loyalty and engagement, with members showing higher purchase frequencies and spending.
Nike’s ability to integrate personalisation across multiple touchpoints—digital and physical—ensures that customers receive a consistent and highly tailored experience. This omnichannel approach not only enhances user engagement but also boosts brand loyalty and sales.
7. ASOS: Advanced Personalisation for Fashion E-commerce
ASOS employs advanced personalisation techniques to create a tailored shopping experience for its users. By analysing user data, including browsing history and purchase behaviour, ASOS offers personalised product recommendations and dynamic content that adapts in real-time.
Key Strategy: Real-Time Data Analysis and Dynamic ContentResults: ASOS’s personalised shopping experience has led to increased user engagement and higher conversion rates.
The use of real-time data analysis allows ASOS to adapt its interface and recommendations instantly, ensuring that users always see the most relevant products. This dynamic approach not only enhances the shopping experience but also drives higher sales and customer satisfaction.
Conclusion
These case studies illustrate the transformative power of personalisation in e-commerce. By leveraging advanced technologies such as AI, machine learning, and AR, along with robust data analytics, these companies have created highly engaging and tailored shopping experiences that drive user satisfaction, loyalty, and sales. The integration of personalisation with SEO strategies ensures that these personalised experiences are discoverable and optimised for visibility, making personalisation not just a user experience enhancer but a key driver of business growth.

Future Trends in Personalised Online Shopping Experiences
The future of personalised online shopping experiences is shaping up to be as thrilling as a plot twist in a best-selling novel. Buckle up as we explore the cutting-edge trends that will redefine how we shop online, blending technology, data, and creativity into a seamless, intuitive, and highly engaging user experience.
Hyper-Personalisation through Advanced AI: If you thought current personalisation was impressive, the future holds even more sophisticated AI-driven personalisation. We're talking about hyper-personalisation, where AI algorithms analyse real-time data points from multiple sources, including social media, browsing history, and even wearable technology, to create a highly individualised shopping experience. This goes beyond recommending products—it involves predicting needs, preferences, and even emotions to offer the most relevant suggestions at the perfect moment. Integrating these AI capabilities with eCommerce SEO ensures that the personalised content is also optimised for visibility.
Voice Commerce: With the rise of smart speakers and voice assistants, voice commerce is set to revolutionise online shopping. Personalised shopping experiences will be driven by conversational AI that understands and anticipates user needs. Imagine simply asking your voice assistant to reorder your favourite brand of coffee, and it not only completes the order but also suggests a new blend you might like based on your past purchases and preferences. Ensuring that voice search optimisation is part of your SEO services strategy will be crucial to capture this growing market.
Augmented Reality (AR) and Virtual Reality (VR): AR and VR are no longer just for gaming—they're becoming integral to online shopping. Personalised AR experiences allow users to "try on" clothes, accessories, or even makeup virtually, tailored to their unique preferences and body measurements. VR takes this a step further, offering immersive virtual shopping environments where users can browse and interact with products in a highly personalised setting. For instance, a furniture retailer could create a VR experience where customers can see how a piece of furniture looks in their home before making a purchase. Incorporating technical SEO ensures these advanced technologies are discoverable by search engines.
Predictive Analytics for Inventory Management: Predictive analytics will play a significant role in not just personalising the shopping experience but also in managing inventory. By analysing trends and customer behaviour, businesses can predict which products will be in demand and when. This ensures that popular items are always in stock, enhancing customer satisfaction. For example, if data indicates a surge in demand for summer wear in a particular region, businesses can stock up accordingly and tailor their advertising services to highlight these products.
Personalised Content Marketing: Content marketing is evolving to be more data-driven and personalised. Future trends will see content that is not only relevant to the user's interests but also tailored to their current context and behaviour. For instance, a user reading about SEO trends might be shown personalised articles, case studies, and even webinars that match their expertise level and industry focus. Employing SEO content writing services ensures this content is also optimised for search engines, driving both engagement and discoverability.
Blockchain for Enhanced Privacy and Personalisation: Blockchain technology offers a promising solution to the privacy concerns associated with personalisation. By allowing users to control their data and decide who can access it, blockchain can create a more secure environment for personalised shopping. Users can share data with confidence, knowing it’s protected, which in turn enables businesses to offer more accurate and personalised recommendations. Integrating blockchain with local SEO services can also enhance transparency and trust in local business transactions.
Real-Time Personalisation: The future will see an increased emphasis on real-time personalisation, where user interfaces adapt instantly based on user actions and preferences. This involves everything from dynamically changing product recommendations to altering the layout of a website in real-time to suit individual user needs. For instance, a user who frequently buys sports equipment might see a homepage tailored to showcase the latest in athletic gear and related articles. Ensuring these dynamic elements are optimised through SEO link building services will be key to maintaining search engine visibility.
Emotional AI and Sentiment Analysis: Understanding and responding to user emotions will be a major trend. Emotional AI can analyse facial expressions, voice tones, and text inputs to gauge a user's mood and adjust the shopping experience accordingly. For example, if a user appears frustrated while browsing a website, the AI can offer live chat support or simplify the interface to improve the experience. Sentiment analysis in product reviews and social media interactions can also inform more personalised marketing strategies, ensuring that the user feels understood and valued.
Sustainable and Ethical Personalisation: As consumers become more conscious of sustainability and ethics, personalisation strategies will need to reflect these values. Personalised recommendations will increasingly highlight sustainable and ethically sourced products, aligning with the user’s values and preferences. For example, an environmentally conscious shopper might receive recommendations for eco-friendly products and brands that align with their values. Integrating these recommendations into Google Ads campaigns can enhance both relevance and engagement.
Integrated Omnichannel Personalisation: The future of personalisation will be seamless across all channels, whether online or offline. An omnichannel approach ensures that personalisation extends beyond the website to include emails, social media, in-store experiences, and even post-purchase interactions. For instance, a user who browses a product online might receive a personalised email with a discount for that product, and if they visit the physical store, the in-store experience would reflect their online preferences. This integration requires robust website services to ensure consistency and coherence across all touchpoints.
In conclusion, the future of personalised online shopping experiences is bright, driven by advances in AI, AR/VR, predictive analytics, and blockchain technology. By staying ahead of these trends and integrating them with robust SEO strategies, businesses can create highly engaging, relevant, and secure shopping experiences that delight users and drive growth.
External references:
- Amazon’s recommendation engine success
- Netflix’s personalised content strategy
- Spotify’s “Discover Weekly” and personalised playlists
- Stitch Fix’s data-driven fashion recommendations
- Sephora’s AI and AR-powered personalised beauty solutions
- Nike’s integrated omnichannel personalisation with NikePlus
- ASOS’s real-time data analysis and dynamic content for personalisation
- Advances in AI for hyper-personalisation in e-commerce
- The impact of voice commerce on online shopping
- Augmented and virtual reality trends in retail
- Predictive analytics for inventory management
- Blockchain technology for enhanced privacy in personalisation