Alright, let's face it, marketers have always had a fascination with the generational divide, much like a child trying to understand the allure of Brussels sprouts. Now, with the power of big data, we've gone from scratching our heads to playing 4D chess. Big data isn't just a buzzword thrown around by tech enthusiasts; it's the secret sauce that allows us to dissect consumer behaviours with surgical precision, much like an overzealous sous-chef at a Michelin-starred restaurant. The goal? To unearth insights that can turbocharge your advertising strategies and craft marketing campaigns that resonate across age groups.
Picture this: you're a marketer armed with a treasure trove of data points. Gen Z's browsing habits, Millennials' spending patterns, Gen X's brand loyalties, and Baby Boomers' purchasing preferences are all at your fingertips. The trick is to sift through this digital goldmine without getting bogged down by analysis paralysis. Enter predictive analytics and machine learning algorithms. These are your trusty sidekicks, helping you make sense of the chaos and draw actionable insights. For instance, leveraging enterprise SEO services can enhance your online presence across multiple generations, ensuring your brand stays relevant and visible.
Now, you might wonder, what exactly can big data tell us about different generations? Well, let's dive into the nitty-gritty. Gen Z, the digital natives, are a curious breed. They value authenticity and are quick to call out brands that fail to walk the talk. Their engagement with brands is heavily influenced by social media trends and influencer endorsements. By analysing social media interactions and sentiment analysis, brands can tailor their messages to appeal to this skeptical yet socially conscious cohort. Additionally, employing SEO content writing techniques that incorporate trending keywords and phrases can capture Gen Z's fleeting attention span.
Millennials, often dubbed the 'experience generation,' are driven by a desire for personalisation and meaningful experiences. They're not just buying products; they're investing in brands that align with their values. Big data helps decode this by tracking purchase history, online behaviour, and engagement metrics. This information can be used to create highly personalised marketing campaigns. Implementing ecommerce SEO strategies ensures that your online store is optimised for the search terms that resonate with Millennials, increasing the chances of conversion.
Gen X, the often-overlooked middle child of generations, exhibits brand loyalty and prefers straightforward, no-nonsense communication. They're the ones who still appreciate a good email newsletter and are more likely to respond to direct marketing efforts. Analysing email open rates, click-through rates, and purchase data can provide valuable insights into their preferences. By using Google Ads effectively, brands can target Gen X with precision, ensuring their messages hit the mark without unnecessary fluff.
Ah, the Baby Boomers. Often seen as the traditionalists, they're not as tech-averse as some might think. In fact, they're increasingly embracing online shopping and social media. Understanding their behaviour through big data involves analysing web traffic, online spending patterns, and social media engagement. Tailoring local SEO strategies to highlight proximity and convenience can effectively attract this demographic, who appreciate both value and ease of access.
So, how does one navigate this labyrinth of data without getting lost? It all boils down to integrating your big data analytics with a robust marketing strategy. This means not just collecting data, but also interpreting and acting on it. By continuously refining your approach based on real-time data, you can stay ahead of consumer trends and preferences. For instance, utilising cost-per-lead estimators can help you budget more effectively and allocate resources where they will have the most impact.
In conclusion, the marriage of big data and marketing is a match made in heaven, provided you know how to harness its power. By understanding multi-generational consumer behaviours through the lens of big data, brands can create more targeted, effective, and resonant marketing campaigns. This not only enhances customer satisfaction but also drives business growth. So, whether you're targeting the Snapchat-savvy Gen Z or the Facebook-friendly Boomers, let big data be your guide in this ever-evolving marketing landscape.
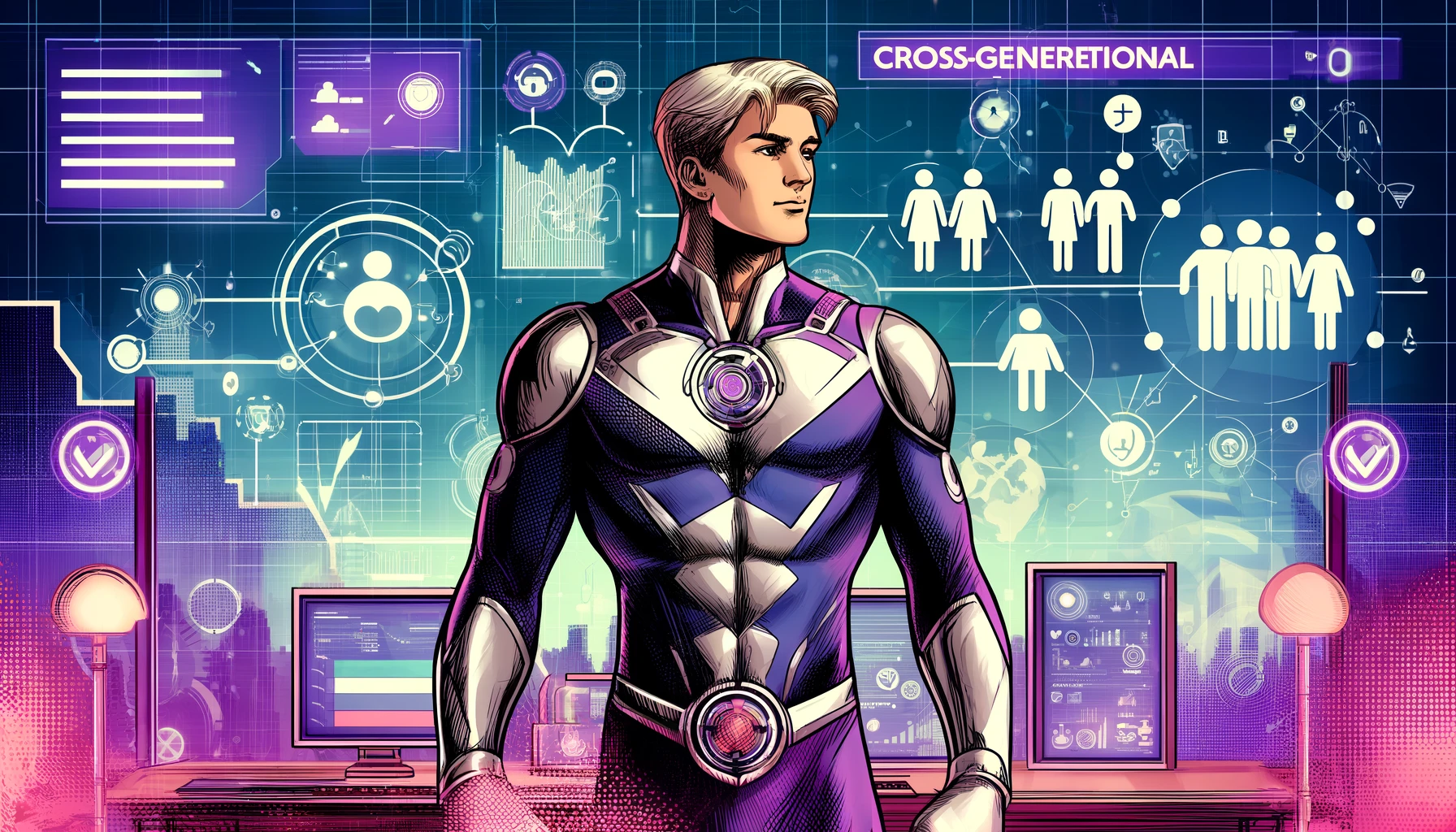
Collecting and Analysing Cross-Generational Data
Alright, buckle up, because diving into the depths of cross-generational data collection is like trying to decipher the enigma machine with a magnifying glass and a monocle. But fear not, as we untangle this data spaghetti, you'll discover insights sharper than a tack and more actionable than a to-do list on a caffeine rush. Cross-generational data isn’t just about lumping together numbers from different age groups; it’s about understanding the unique quirks, preferences, and behaviours that make each generation tick.
First, let’s talk collection. To get a comprehensive view of cross-generational behaviours, one must employ a multi-channel approach. Think of it as casting a wide net to catch the most diverse set of fish in the data ocean. Social media analytics, website traffic data, purchase histories, email open rates, and even IoT device interactions provide a wealth of information. The trick lies in integrating these disparate data sources into a unified data management platform. This platform acts as the central nervous system, processing and analysing data streams in real-time.
Social media platforms are gold mines for generational data. Gen Z, for instance, practically live on platforms like TikTok and Instagram, providing rich veins of data through their engagement patterns, hashtag usage, and content preferences. Millennials, while also active on social media, show distinct patterns on platforms like Facebook and Twitter. By leveraging advanced advertising services, marketers can segment audiences and tailor campaigns that resonate with the specific interests of each generation.
Now, you can’t discuss data collection without mentioning cookies – not the chocolate chip kind, although they do make data crunching more enjoyable. Web cookies track user activity across websites, creating detailed profiles of browsing habits. This is particularly useful for understanding the more elusive Gen X and Baby Boomer cohorts, who may not be as vocal on social media but leave digital footprints wherever they go online. Integrating these insights with technical SEO strategies can significantly improve search engine rankings and user engagement across all age groups.
But data collection is only half the battle; the real magic happens during analysis. This is where machine learning algorithms and AI come into play, transforming raw data into actionable insights. Predictive analytics can forecast future behaviours by identifying patterns and trends within the data. For example, by analysing past purchase behaviours, one can predict the likelihood of Gen Z adopting a new tech gadget or Millennials splurging on a travel experience. Implementing website services that include advanced analytics tools can help businesses stay ahead of the curve.
Segmentation is another critical aspect of data analysis. By dividing the data into generational cohorts, businesses can identify specific trends and preferences unique to each group. For instance, while Gen Z may show a penchant for sustainable and eco-friendly products, Baby Boomers might prioritise quality and brand heritage. This granular level of detail allows for more targeted marketing campaigns. Employing SEO link building can further enhance these efforts by driving high-quality traffic from relevant sources, ensuring that the right message reaches the right audience.
Data visualisation tools are essential in this process, turning complex datasets into easily digestible graphs and charts. This not only aids in understanding but also in communicating insights to stakeholders. Interactive dashboards can provide real-time updates, highlighting shifts in consumer behaviour as they happen. For example, a spike in online searches for a particular product among Millennials could prompt an immediate marketing push. Integrating WordPress website services with these tools ensures seamless updates and robust performance.
Finally, let’s not forget the ethical considerations. With great data comes great responsibility. Ensuring data privacy and adhering to regulations like GDPR is paramount. Transparent data practices build trust with consumers, who are increasingly aware and concerned about how their data is used. A comprehensive approach to data security and privacy is not just a legal obligation but a competitive advantage in today’s data-driven market.
In conclusion, collecting and analysing cross-generational data is a sophisticated endeavour that requires a blend of advanced technology, strategic thinking, and ethical considerations. By leveraging multi-channel data collection, employing cutting-edge analytics, and maintaining a focus on segmentation and privacy, businesses can unlock the full potential of their data. This not only drives more effective marketing campaigns but also fosters deeper connections with consumers across generations, ensuring long-term success and loyalty.
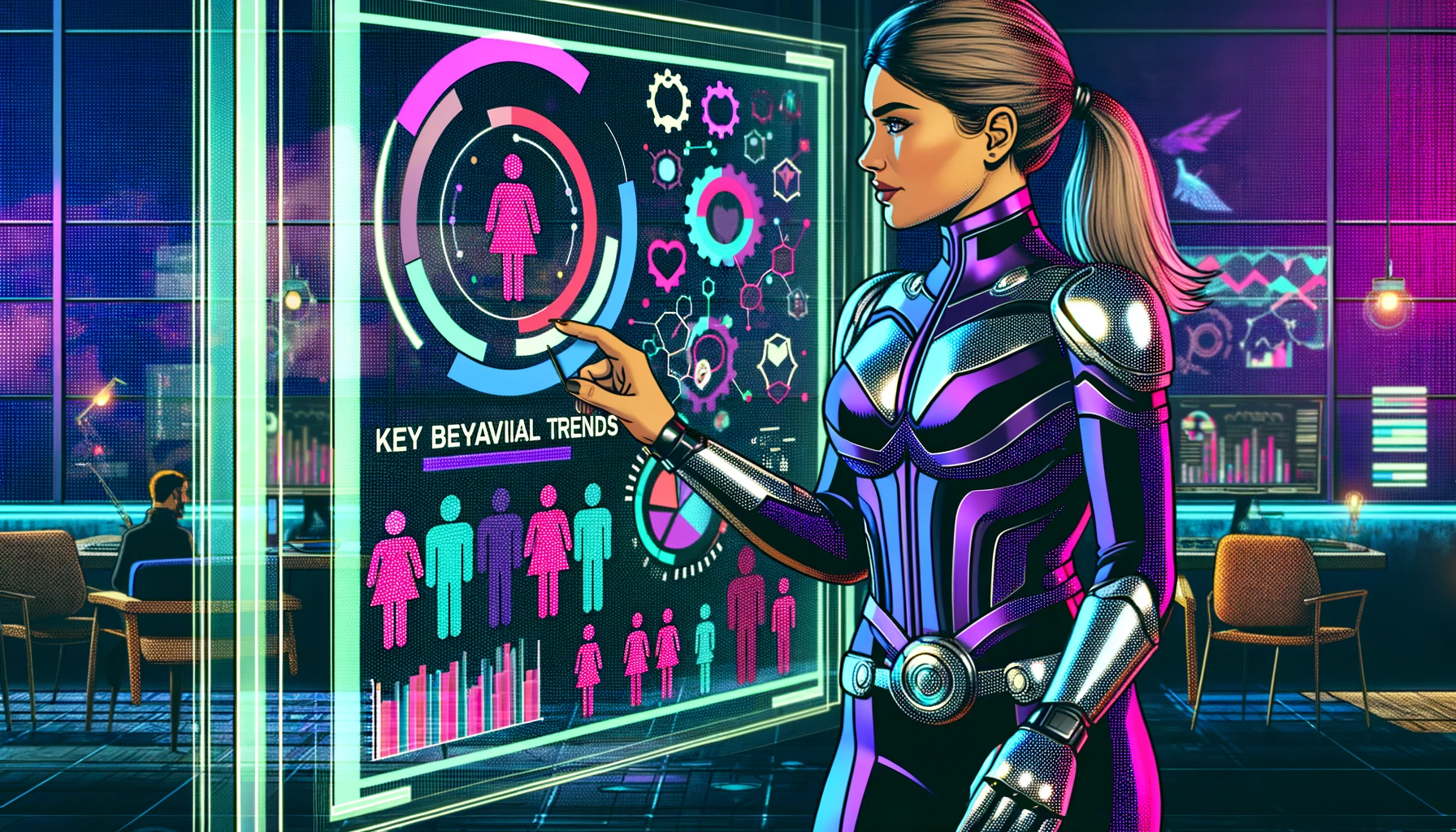
Identifying Key Behavioural Trends Across Age Groups
Let's dive straight into the tantalising world of generational behavioural trends, a realm where marketers play detective to uncover the intricate patterns that define each age group's interaction with brands and technology. By leveraging big data and advanced analytics, we can peel back the layers to reveal what truly makes Gen Z, Millennials, Gen X, and Baby Boomers tick. It's like being a fly on the wall at a multigenerational family reunion, but without the risk of getting swatted.
First up, let's talk about the digital darlings: Gen Z. Born with smartphones in their hands and a scepticism for traditional advertising, this cohort thrives on authenticity and engagement. They value brands that align with their social and environmental values and are quick to endorse or call out brands based on their ethical stances. Data from social media platforms like TikTok and Instagram reveal that influencer marketing and user-generated content resonate profoundly with this group. Brands can harness this by implementing Instagram ads strategies that feature real people and genuine experiences, making the most of their propensity for sharing and creating viral content.
Millennials, often branded as the 'experience generation,' show a distinct preference for personalised experiences and brands that offer more than just a product. They are avid users of social media but also rely heavily on peer reviews and recommendations. Behavioural data shows that they are more likely to engage with brands that provide a seamless omnichannel experience. By utilising ecommerce SEO techniques, businesses can ensure that their online platforms offer personalised shopping experiences, leveraging data to recommend products and create targeted promotions that align with Millennial values and interests.
Now, let's move to Gen X, the often-overlooked middle child of the generational spectrum. They are known for their brand loyalty and preference for value and quality. Gen Xers are more likely to respond to email marketing and traditional digital advertising than their younger counterparts. They appreciate clear, concise communication and reliable customer service. Analysing their behaviour through CRM systems and email campaign metrics can provide insights into their purchasing habits and brand interactions. Employing Google Ads to target this demographic can be highly effective, especially when ads are tailored to highlight product durability and long-term value.
Finally, we have the Baby Boomers, the generation that still remembers life before the internet but has adapted remarkably well to the digital age. They tend to have higher disposable incomes and are often loyal to brands they trust. This group values convenience and straightforwardness, making them more likely to engage with well-designed websites and easy-to-navigate online stores. Big data reveals that they respond well to digital newsletters and online reviews. By optimising local near me SEO, businesses can ensure that Baby Boomers find their services easily, promoting trust and convenience.
Now, let's synthesise these insights into actionable strategies. Understanding that Gen Z values social proof and authenticity, brands should invest in creating compelling stories and leveraging influencers who genuinely align with their values. Millennials, with their craving for unique experiences, respond best to personalised marketing efforts. Use data analytics to track their interactions and craft bespoke offers that cater to their preferences. For Gen X, it's all about reliability and value. Highlight these aspects in your marketing messages and ensure that your customer service is top-notch. Baby Boomers, while tech-savvy, appreciate simplicity and direct communication. Make sure your digital platforms are user-friendly and your marketing messages are clear and benefit-focused.
Integrating these strategies with robust data analytics platforms allows brands to adapt in real-time. Predictive analytics can forecast trends and consumer shifts, helping businesses stay ahead of the curve. For instance, if data indicates a growing trend among Millennials for sustainable products, brands can adjust their inventory and marketing strategies accordingly.
In conclusion, identifying key behavioural trends across age groups is not just about gathering data; it’s about interpreting it through the lens of each generation's unique characteristics and preferences. By leveraging advertising services tailored to these insights, brands can create more effective and resonant marketing campaigns. This approach ensures that marketing efforts are not only relevant but also deeply engaging, fostering brand loyalty and driving business growth across all generational cohorts.

Customising Marketing Strategies for Generational Preferences
Alright, let's cut to the chase—generational marketing is like playing a high-stakes game of chess where each move needs to be tailored to the unique preferences of your opponent. Understanding and addressing the distinct characteristics of Gen Z, Millennials, Gen X, and Baby Boomers requires a blend of data-driven insights and creative finesse. Let's dive into how to customise your marketing strategies to captivate each generation, turning every campaign into a checkmate.
Starting with Gen Z, the digital natives who are as elusive as a cat meme at a dog show. This cohort is known for its short attention span, high social media engagement, and a strong preference for authenticity and transparency. To hook this savvy audience, brands need to be where they are: TikTok, Instagram, and Snapchat. It's not just about being present, though; it's about resonating. Gen Z values user-generated content and influencer partnerships. Implementing Instagram ads that showcase real people and genuine experiences can build trust and drive engagement. Additionally, integrating ecommerce SEO to ensure your online presence is optimised for mobile can capture this generation's attention where they spend most of their time.
Millennials, on the other hand, are the kings and queens of personalisation. They expect brands to know them intimately, anticipating their needs and desires. This generation is heavily influenced by peer reviews and social proof. Crafting personalised marketing campaigns that leverage data analytics can make a world of difference. For instance, using Google Ads to retarget users based on their browsing history or previous purchases can create a more personalised shopping experience. Moreover, brands should focus on creating memorable experiences, whether through immersive digital content or unique in-store interactions. Implementing website services that offer seamless and personalised user experiences can significantly boost Millennial engagement.
Moving to Gen X, the generation that often flies under the marketing radar but holds substantial purchasing power. Known for their brand loyalty and practicality, Gen Xers appreciate straightforward communication and value-driven offers. Email marketing and direct mail are particularly effective for this group. Utilising CRM data to send targeted email campaigns that highlight product durability and long-term benefits can resonate well. Additionally, incorporating technical SEO to ensure your content is easily accessible and clearly communicates your value proposition can enhance your reach and impact with this demographic.
Then we have the Baby Boomers, who are often perceived as the most traditional yet are surprisingly tech-savvy. They value quality, customer service, and straightforwardness. For this group, a well-designed, easy-to-navigate website is crucial. Brands should focus on local near me SEO to ensure Boomers can easily find nearby services and products. Moreover, leveraging social media platforms like Facebook, where Boomers are quite active, can enhance engagement. Direct and clear messaging that emphasises product quality and reliable customer service can build trust and loyalty among this generation.
To summarise, customising marketing strategies for generational preferences requires a deep understanding of each group's unique characteristics and behaviours. For Gen Z, it's all about authenticity and social media engagement; for Millennials, personalisation and experience are key; Gen X values straightforward communication and reliability; and Baby Boomers prioritise quality and simplicity. By leveraging advanced data analytics and targeted advertising services, brands can create campaigns that not only reach but resonate with each generation.
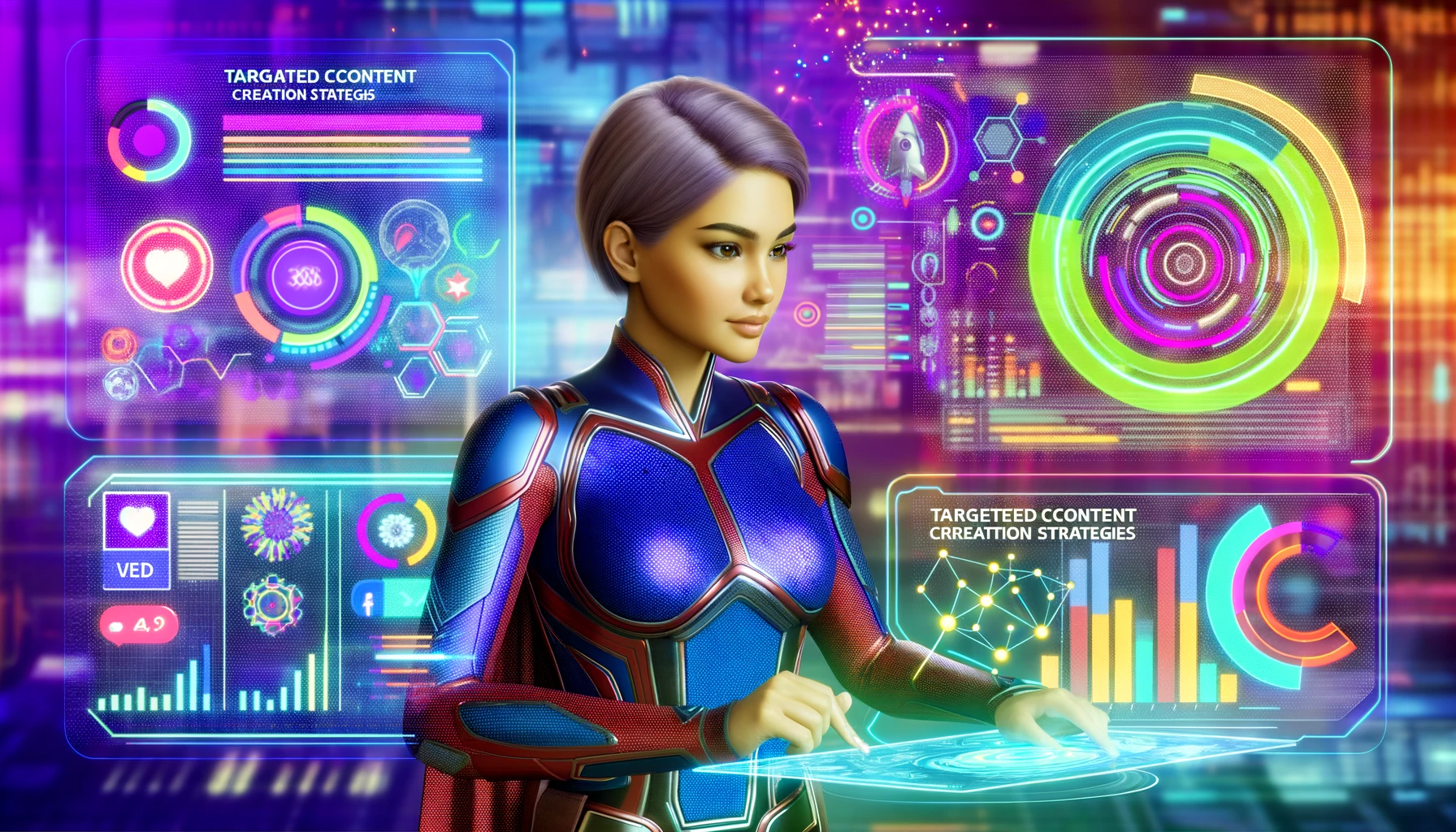
Leveraging Big Data for Targeted Content Creation
Alright, let’s dive into the data vortex and unravel how leveraging big data can transform targeted content creation from a guessing game into a precision-guided missile. Big data isn't just a term for impressing at dinner parties; it’s the bedrock of modern marketing strategies, especially when it comes to crafting content that hits the sweet spot for your audience. Let's break down how to harness this powerhouse to create content that speaks directly to different segments of your audience.
First things first, the raw materials. Big data encompasses a vast array of information from diverse sources: social media interactions, browsing behaviours, purchase histories, and even IoT data. The key is to collect and integrate this data into a cohesive platform. By utilising sophisticated data management systems, brands can sift through this digital haystack to find the needles of insight. For example, analysing social media data can reveal trending topics and consumer sentiments, enabling brands to craft timely and relevant content. Employing advanced advertising services can facilitate the collection and analysis of this data, ensuring your campaigns are always data-driven.
Now, let's get into the specifics of how big data informs targeted content creation. Consider social media platforms like Twitter and Facebook. They provide a wealth of user data, from demographic information to real-time engagement metrics. By analysing this data, brands can identify the most effective times to post, the types of content that generate the most engagement, and the topics that resonate with different audience segments. This granular level of detail allows for the creation of content that is not only relevant but also highly engaging. Implementing SEO content writing strategies that incorporate these insights can significantly boost your content’s visibility and impact.
Understanding the preferences and behaviours of different generational cohorts is another critical aspect. For instance, Gen Z’s penchant for short, visually engaging content means that brands should focus on creating bite-sized videos and infographics. Analysing data from platforms like TikTok can provide insights into what types of content are trending among this group. On the other hand, Millennials, who value in-depth information and personalisation, might prefer long-form articles and interactive content. Using website services that allow for the creation of customised content experiences can help cater to these preferences.
Segmentation is where big data truly shines. By dividing your audience into specific segments based on their behaviours, preferences, and demographics, you can create highly targeted content. For example, purchase history data can reveal which products are most popular among certain age groups or geographic locations. This information can be used to create content that highlights these products, tailored to the specific interests of each segment. Employing ecommerce SEO ensures that this content reaches the right audience at the right time, driving higher engagement and conversion rates.
Predictive analytics, a subset of big data, can also play a pivotal role in content creation. By analysing past behaviours, predictive models can forecast future trends and consumer needs. This allows brands to stay ahead of the curve by creating content that anticipates these trends. For instance, if predictive analytics indicate a rising interest in sustainable products among Millennials, brands can produce content that highlights their eco-friendly initiatives. Integrating these insights with technical SEO strategies can enhance your content’s discoverability, ensuring it reaches a wider audience.
Lastly, let’s not forget the importance of real-time data. In today’s fast-paced digital landscape, staying relevant means being able to adapt quickly to changing trends and consumer interests. Real-time data analytics can provide immediate insights into how your content is performing, allowing you to make quick adjustments and optimise your strategy on the fly. This agility is crucial for maintaining engagement and staying ahead of competitors. Leveraging cost-per-lead estimators can help allocate your budget more effectively, ensuring you get the most bang for your buck with your content marketing efforts.
In conclusion, leveraging big data for targeted content creation is about more than just collecting information; it’s about transforming that information into actionable insights that drive engagement and conversion. By understanding the unique preferences and behaviours of different audience segments, employing advanced analytics, and staying agile with real-time data, brands can create content that truly resonates. This data-driven approach not only enhances the effectiveness of your marketing campaigns but also fosters deeper connections with your audience, driving long-term loyalty and growth.
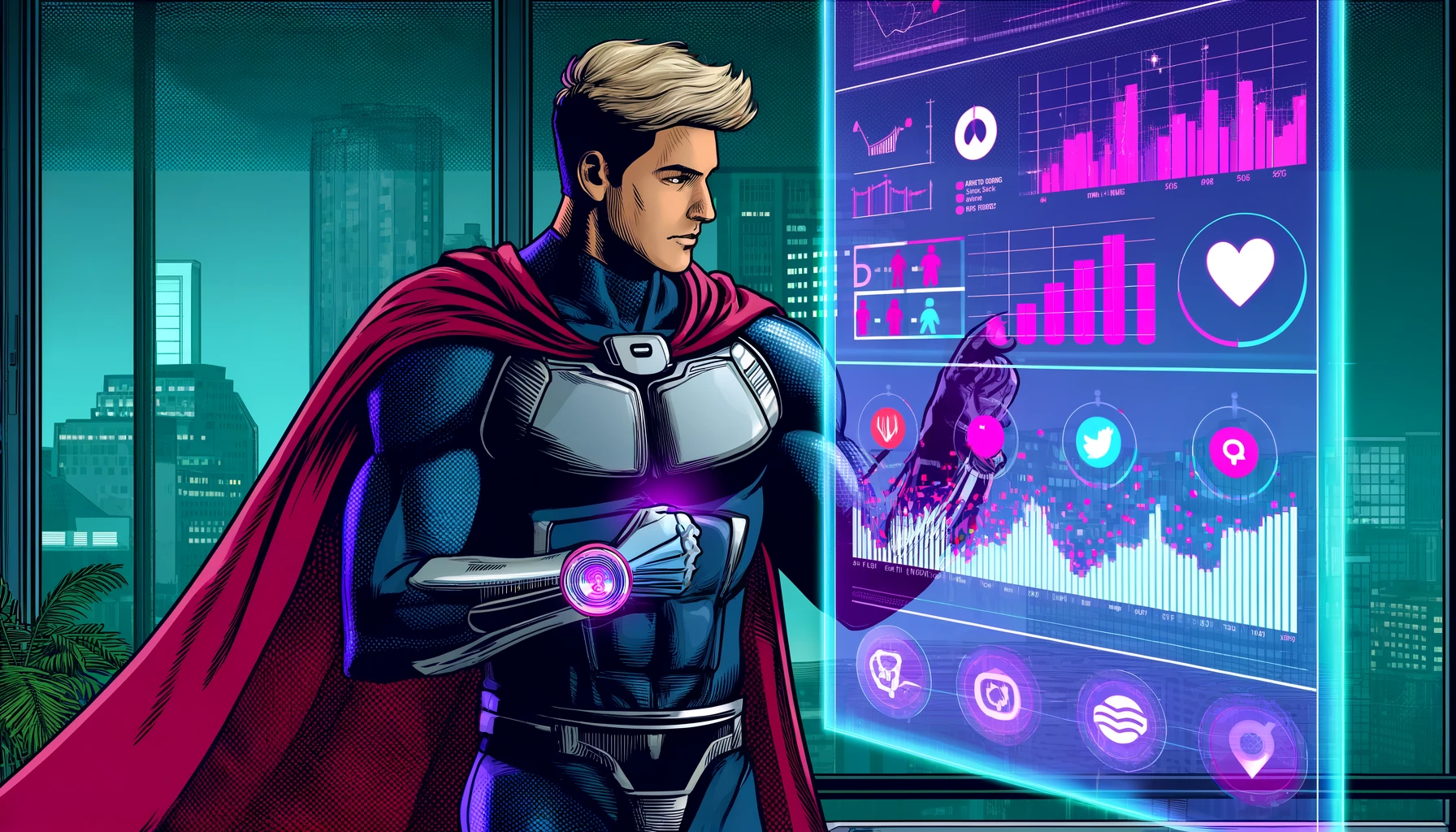
Predicting Consumer Behaviour with Data Analytics
Alright, let's dive into the crystal ball of the digital age—data analytics. Predicting consumer behaviour isn't about peering into a murky cauldron; it's about harnessing the vast power of data to make informed, strategic decisions. With advanced analytics, we can transform raw data into actionable insights, giving brands the edge in anticipating and responding to consumer needs and trends. Let's unpack how data analytics can predict consumer behaviour with the precision of a Swiss watch.
Firstly, let's talk about the types of data at our disposal. Consumer data comes from myriad sources: social media interactions, transaction histories, website analytics, customer feedback, and even IoT devices. This diverse data pool provides a 360-degree view of consumer behaviour, preferences, and trends. The challenge lies in integrating these disparate data points into a cohesive analytics platform. By employing sophisticated data management tools, businesses can aggregate and analyse this data to uncover hidden patterns and correlations.
Machine learning algorithms are the workhorses of predictive analytics. These algorithms can process vast amounts of data at lightning speed, identifying patterns and trends that would be impossible for humans to detect. For example, by analysing past purchasing behaviours, machine learning models can predict future buying patterns with remarkable accuracy. This predictive capability allows brands to tailor their marketing strategies to individual consumers, creating highly personalised experiences. Integrating advertising services that utilise these algorithms can significantly enhance the effectiveness of targeted campaigns.
Consider the case of recommendation engines, which are a staple of e-commerce giants like Amazon and Netflix. These engines use predictive analytics to suggest products or content based on past behaviours and preferences. By analysing browsing history, purchase records, and even time spent on particular product pages, these engines can predict what consumers are likely to buy next. Implementing ecommerce SEO strategies that incorporate such recommendation engines can drive higher engagement and conversion rates by presenting consumers with highly relevant suggestions.
Predictive analytics also shines in customer segmentation. By dividing the consumer base into distinct segments based on behavioural data, brands can develop tailored marketing strategies for each group. For instance, clustering algorithms can group consumers by their purchasing habits, engagement levels, or product preferences. This segmentation allows for more precise targeting and personalised communication. Using SEO content writing techniques that address the specific interests of each segment can enhance content relevance and boost SEO performance.
Another powerful application of predictive analytics is in churn prediction. By analysing factors such as purchase frequency, engagement metrics, and customer feedback, predictive models can identify which customers are at risk of churning. This insight allows brands to take proactive measures to retain these customers, such as offering personalised incentives or improving customer support. Employing website services that integrate these predictive tools can enhance customer retention strategies and reduce churn rates.
Let's not overlook the role of sentiment analysis in predicting consumer behaviour. By analysing social media posts, reviews, and other forms of user-generated content, sentiment analysis tools can gauge consumer sentiment towards a brand or product. This real-time feedback is invaluable for predicting how consumers will respond to new products, marketing campaigns, or changes in service. Implementing technical SEO strategies that optimise content for sentiment analysis can improve the accuracy of these predictions and enhance overall consumer engagement.
The real-time nature of predictive analytics cannot be overstated. In today's fast-paced digital landscape, being able to respond to trends and consumer behaviour in real-time is a significant competitive advantage. Real-time data analytics tools provide immediate insights into consumer actions, allowing brands to adjust their strategies on the fly. For instance, if a sudden spike in interest for a particular product is detected, brands can quickly ramp up marketing efforts for that product, ensuring they capitalise on the trend. Leveraging cost-per-lead estimators can help allocate resources more effectively, maximising the ROI of marketing campaigns.
In conclusion, predicting consumer behaviour with data analytics is about transforming data into actionable insights that drive strategic decision-making. By leveraging machine learning, predictive models, and real-time analytics, brands can anticipate consumer needs and trends with unprecedented accuracy. This not only enhances the effectiveness of marketing campaigns but also fosters deeper, more personalised connections with consumers, driving long-term loyalty and growth.
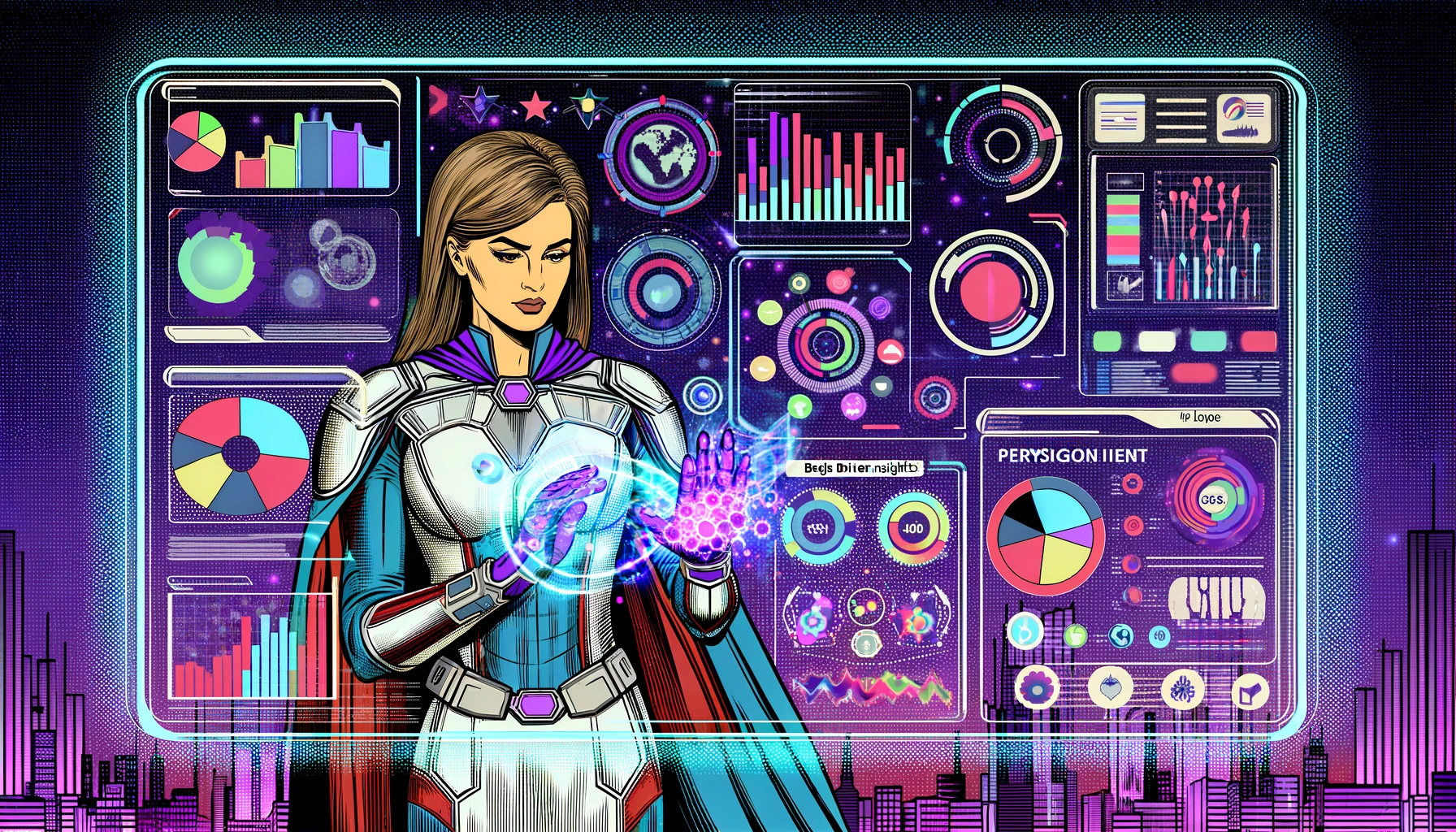
Integrating Big Data Insights with Personalisation Engines
Alright, let’s dive into the nuts and bolts of integrating big data insights with personalisation engines, a process akin to creating a bespoke suit for each customer but at a digital scale. This integration transforms how brands interact with consumers, turning data-driven insights into hyper-personalised experiences that resonate on a deeply individual level.
Firstly, understanding the role of big data in personalisation is crucial. Big data provides a comprehensive view of consumer behaviour, preferences, and trends by aggregating information from various sources such as social media, transaction records, browsing histories, and even IoT device data. This wealth of information is the raw material that personalisation engines use to tailor experiences to individual users. To effectively harness this data, businesses need robust data management systems capable of processing and analysing vast amounts of information in real-time.
Personalisation engines, the brain behind tailored experiences, use advanced algorithms and machine learning models to analyse big data and predict consumer preferences. These engines can dynamically adjust content, product recommendations, and marketing messages to align with individual user profiles. For instance, an ecommerce site leveraging ecommerce SEO and big data insights can provide personalised product suggestions based on a user’s past purchases, browsing history, and even similar users' behaviours. This not only enhances user engagement but also significantly boosts conversion rates.
Let's look at some practical applications. Recommendation engines, a subset of personalisation engines, are a perfect example of how big data integration works in practice. By analysing patterns in consumer behaviour, these engines can suggest products or content that are most likely to appeal to individual users. This technique is famously used by platforms like Amazon and Netflix, which rely heavily on advertising services that incorporate recommendation algorithms to enhance user satisfaction and retention. By predicting what users are most likely to buy or watch next, these platforms ensure a personalised experience that keeps users coming back for more.
Another critical application is personalised email marketing. By integrating big data insights into email marketing platforms, businesses can create highly targeted email campaigns. These campaigns can be tailored based on various factors such as past purchase behaviour, browsing history, and even the time of day when a user is most likely to open their emails. For instance, leveraging SEO content writing to craft personalised email content that resonates with individual preferences can lead to higher open rates, click-through rates, and ultimately, conversions.
Moreover, integrating big data with personalisation engines extends beyond ecommerce and email marketing. Websites and mobile apps can dynamically adjust their content and layout to match user preferences in real-time. For example, a news website can personalise its homepage based on a user’s reading habits, showing more articles on topics they are interested in. Implementing website services that incorporate these personalisation capabilities can enhance user experience and engagement, making the digital interaction more intuitive and enjoyable.
The role of predictive analytics in personalisation cannot be overstated. Predictive models analyse historical data to forecast future behaviour, allowing personalisation engines to preemptively adjust content and recommendations. For instance, if predictive analytics indicate a rising interest in eco-friendly products among a segment of users, the personalisation engine can highlight these products to the relevant users. Integrating technical SEO with predictive analytics ensures that the content is not only personalised but also optimised for search engines, enhancing visibility and reach.
One of the most significant benefits of integrating big data insights with personalisation engines is the ability to deliver real-time personalisation. This means that as users interact with a brand’s digital platforms, the content and recommendations they see can be adjusted on the fly. Real-time data analytics tools provide immediate feedback on user actions, allowing for dynamic content adjustments that enhance the user experience. This level of responsiveness is critical in today’s fast-paced digital landscape, where consumer preferences can shift rapidly. Leveraging cost-per-lead estimators can help allocate resources effectively, ensuring that marketing efforts are both targeted and efficient.
In conclusion, integrating big data insights with personalisation engines is a game-changer for creating tailored, engaging, and effective consumer experiences. By leveraging the power of big data, businesses can understand their customers on a deeper level and deliver personalised content that meets their unique needs and preferences. This not only drives engagement and conversions but also fosters long-term loyalty and satisfaction, ensuring sustained business growth in a competitive market.
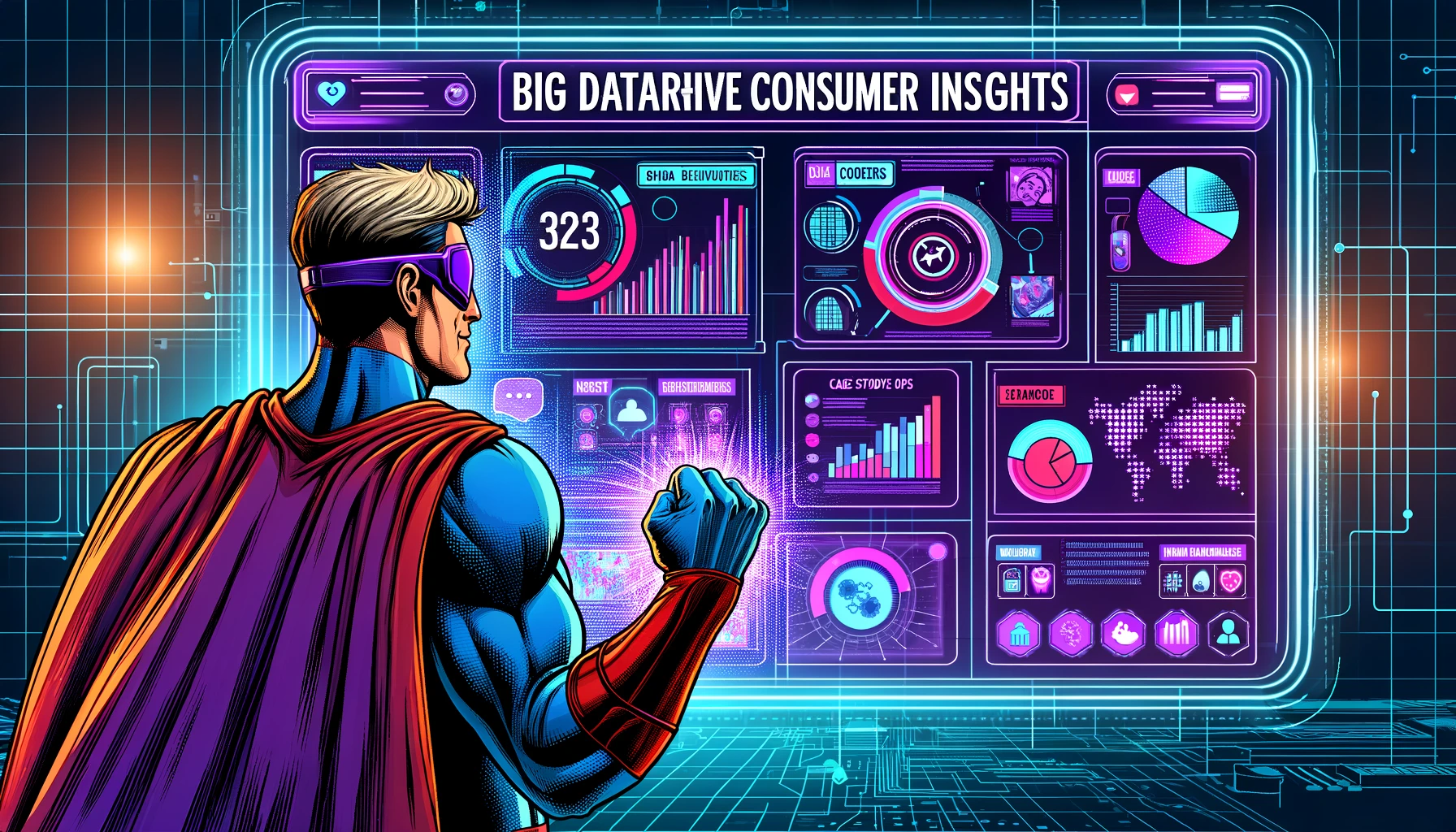
Case Studies of Big Data-Driven Consumer Insights
Let’s dive into the fascinating world of case studies where big data-driven consumer insights have led to game-changing strategies and innovations. These examples demonstrate the transformative power of big data, from boosting customer engagement to driving unprecedented growth.
Netflix: Predictive Analytics for Content Creation
Netflix is the poster child for leveraging big data to deliver personalised user experiences. By analysing the viewing habits of its 223 million subscribers, Netflix can predict what content will be popular. The data includes viewing times, content preferences, user ratings, and even the times users pause, rewind, or fast-forward. This wealth of information enabled Netflix to make data-driven decisions in content creation, exemplified by the production of "House of Cards". By analysing that users who watched political dramas also enjoyed content featuring Kevin Spacey and David Fincher, Netflix greenlit the series, which became a massive hit. Implementing advanced advertising services helped Netflix not only promote "House of Cards" effectively but also refine their recommendation algorithms, driving higher engagement and viewer retention.
Amazon: Personalised Shopping Experience
Amazon uses big data to create an incredibly personalised shopping experience. Through its recommendation engine, Amazon analyses user browsing history, purchase history, wish lists, and even items left in the shopping cart. This data allows Amazon to suggest products that users are likely to buy, significantly increasing sales. The system also takes into account what similar users have purchased, refining the recommendations further. This personalised approach is powered by complex algorithms and machine learning, ensuring that the recommendations are relevant and timely. By integrating ecommerce SEO, Amazon ensures that their product listings are optimised for search engines, driving both visibility and conversions.
Spotify: Enhancing User Experience with Personalisation
Spotify’s use of big data is a masterclass in creating a personalised user experience. By analysing data points such as song preferences, listening times, playlist creation, and user interactions, Spotify can tailor its recommendations to individual users. The "Discover Weekly" playlist is a prime example, where each user receives a unique playlist every week based on their listening habits and the habits of users with similar tastes. This feature has significantly increased user engagement and satisfaction. Integrating website services that support real-time data processing allows Spotify to continually refine its recommendations, keeping users hooked.
Target: Predictive Analytics for Customer Insights
Target famously used predictive analytics to identify customers who were likely to be pregnant before they had announced it. By analysing purchasing patterns, such as increased purchases of unscented lotion and vitamin supplements, Target’s data scientists could predict a customer’s pregnancy and send them targeted promotions for baby products. This case demonstrated both the power and the ethical considerations of big data analytics. Target's approach involved sophisticated models that segmented their customer base and personalised marketing efforts accordingly. Employing technical SEO ensured that Target’s online content reached the right audience, driving engagement and sales.
Coca-Cola: Social Media Analytics for Brand Engagement
Coca-Cola leverages big data to monitor and engage with consumers on social media. By analysing millions of social media posts, Coca-Cola can identify trends, sentiments, and preferences in real-time. This allows the company to tailor its marketing campaigns and product offerings to meet the changing demands of its customers. For instance, Coca-Cola used data from social media to inform its "Share a Coke" campaign, which featured personalised bottles with popular names. This campaign significantly boosted sales and brand engagement. Implementing SEO content writing strategies that reflect current trends and consumer sentiments ensures that Coca-Cola’s online presence remains vibrant and engaging.
Starbucks: Optimising Customer Experience with Data
Starbucks utilises big data to enhance the customer experience through its loyalty program and mobile app. By analysing transaction data, customer preferences, and purchasing patterns, Starbucks can personalise offers and recommendations. The mobile app uses this data to send personalised notifications about promotions and new products, driving both engagement and sales. Additionally, Starbucks employs location data to optimise store operations and improve the customer experience. Integrating cost-per-lead estimators helps Starbucks allocate marketing resources efficiently, ensuring maximum ROI.
In conclusion, these case studies highlight the transformative impact of big data-driven consumer insights. From personalising user experiences to predicting customer needs, big data enables brands to make informed decisions that drive engagement, satisfaction, and growth. By leveraging advanced data analytics, businesses can stay ahead of the competition, creating tailored strategies that resonate deeply with their audience.
References
- Enterprise SEO Services
- SEO Content Writing
- Ecommerce SEO
- Google Ads
- Local SEO Strategies
- Cost-per-Lead Estimator