Imagine your website is a guest at a dinner party – now, if it starts talking about itself non-stop, guests (visitors) will quickly make a beeline for the exit. But, if it engages each guest with personalised, intriguing conversations, well, that's a party everyone will remember. Welcome to the AI-powered personalisation fiesta, where conversion rates soar like a well-mixed cocktail.
AI-powered personalisation isn't just about slapping a user's name on a webpage. It's the art of using machine learning algorithms to analyse user behaviour, preferences, and real-time interactions to tailor experiences that are as unique as a bespoke suit. By integrating advanced data analytics, AI discerns patterns and trends from user data, deploying strategies that can predict and influence consumer behaviour more precisely than a crystal ball.
Consider this: a visitor lands on your site, and AI kicks into action, analysing past behaviour and similar user profiles. It might serve up a customised homepage that highlights products or content most likely to appeal. This dynamic tailoring extends to product recommendations, targeted content, and even personalised pricing strategies. It's like having a digital butler, ready to serve exactly what your visitor wants before they even know they want it.
Let's not overlook the power of predictive analytics. By evaluating historical data, AI can anticipate future actions, presenting timely offers that convert indecisive browsers into buyers. This isn't just smart; it's Sherlock Holmes in a server, deducing desires and needs with uncanny accuracy.
AI-driven chatbots also play a pivotal role in personalisation, providing instant customer service that's responsive and tailored to individual queries. They can track interaction history, ensuring conversations pick up right where they left off, creating a seamless user experience that builds trust and drives conversions. Now, if only real-life conversations could be so effortlessly picked up!
Integrating these AI technologies requires an underlying robust data infrastructure and often collaboration with a technical SEO team to ensure the website can handle the complex algorithms and data processing. It's essential that this infrastructure supports real-time data processing, enabling immediate personalisation adjustments that enhance user experience on the fly.
Moreover, incorporating AI-powered personalisation into e-commerce SEO strategies is akin to turbocharging your engine. By leveraging AI, you can optimise product listings, descriptions, and meta tags based on the latest user search trends and behaviour patterns, driving more organic traffic to the site and enhancing conversion rates.
But don't just take my word for it. According to a study by Epsilon, 80% of consumers are more likely to make a purchase when brands offer personalised experiences. Another report by McKinsey highlights that personalisation can reduce acquisition costs by as much as 50%, lift revenues by 5 to 15%, and increase the efficiency of marketing spend by 10 to 30%. Clearly, this isn't just a fleeting trend; it's a seismic shift in digital marketing strategy.
Yet, implementing AI personalisation isn't without its challenges. Data privacy concerns loom large, with regulations such as GDPR demanding meticulous attention to how user data is collected, stored, and used. Ensuring transparency and gaining user consent is not just a legal obligation but a trust-building exercise crucial for long-term success.
In summary, AI-powered personalisation transforms websites from static displays into dynamic, engaging experiences that anticipate and meet user needs with precision. It involves intricate data analysis, predictive modelling, and real-time adjustments, supported by a robust technical infrastructure. As AI continues to evolve, those who harness its full potential will undoubtedly lead the charge in digital marketing, leaving their competitors trying to catch up.
So, when considering how to enhance your website's conversion rates, remember: it's all about making your digital guest feel seen, heard, and valued. The more personal the experience, the more likely they are to stick around and, crucially, convert.
For those ready to leap into the future of personalised marketing, you might consider exploring our Google Ads services for targeted ad campaigns, or delve into SEO content writing to craft compelling, personalised content that resonates with your audience.
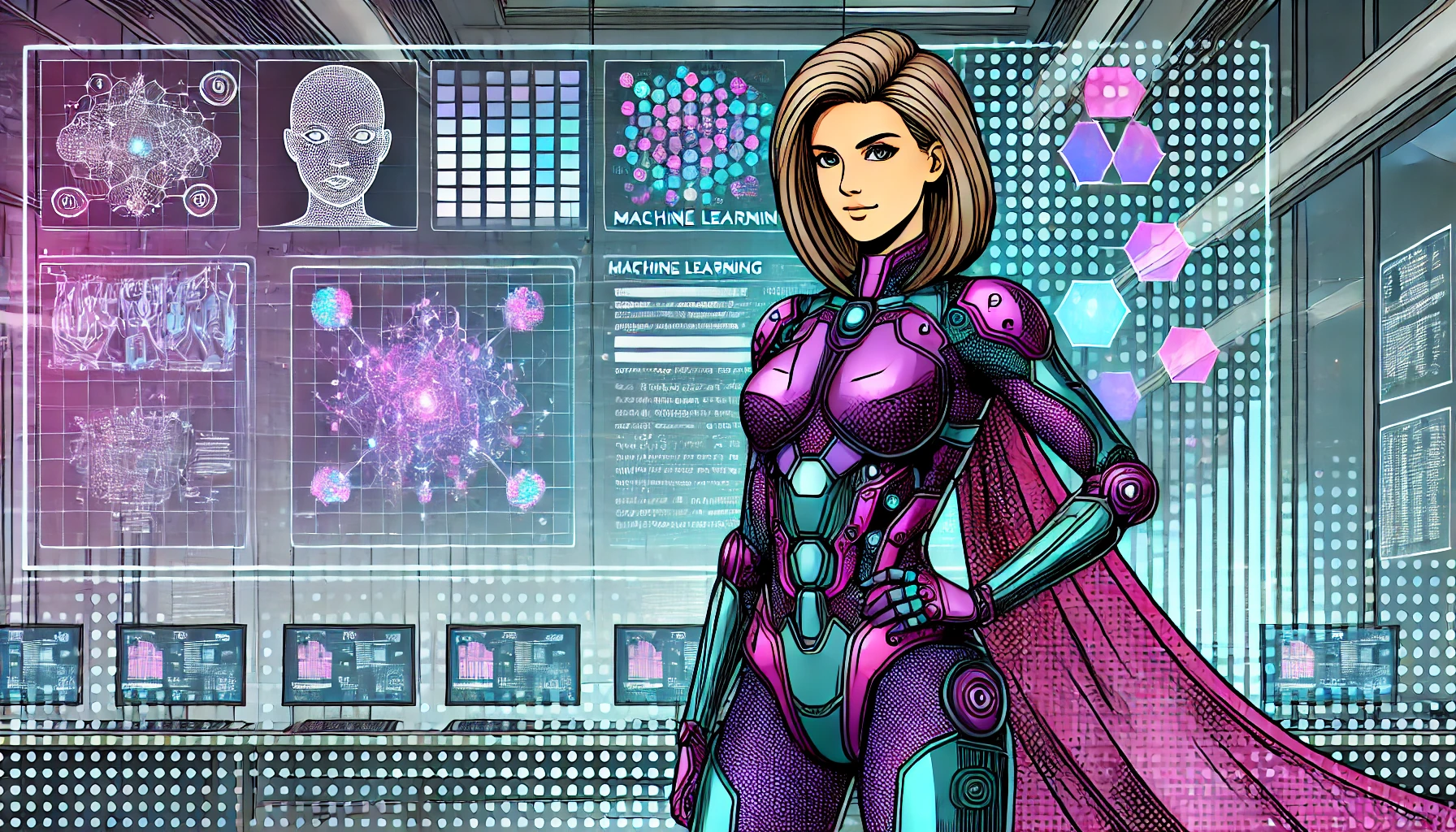
Leveraging Machine Learning Algorithms for Personalisation
Machine learning algorithms are the secret sauce behind the kind of personalisation that makes users feel like a website has rolled out the red carpet just for them. But let's not get ahead of ourselves; this isn't your typical recommendation engine. We're talking about algorithms that learn from every click, hover, and scroll to craft experiences as unique as a snowflake in a blizzard.
The power of machine learning lies in its ability to analyse vast amounts of data and identify patterns faster than a caffeinated coder. Imagine a user browsing your website. Every interaction, from the pages they visit to the products they linger over, feeds into the machine learning models. These algorithms, with a bit of data magic, can predict what the user wants to see next, serving up content and product suggestions that feel almost telepathic.
One of the most potent tools in this arsenal is collaborative filtering. This algorithm analyses user behaviour to recommend items based on what similar users have interacted with. Think of it as the digital equivalent of peer pressure, but in a good way. If User A loves vintage vinyl and User B, who also loves vintage vinyl, buys a new release, User A will likely see that new release on their homepage. It's no coincidence; it's collaborative filtering at work.
Then there's content-based filtering, which takes a different approach. Instead of looking at what similar users do, it analyses the characteristics of items a user has interacted with to recommend similar items. If you've spent an hour reading articles about the benefits of organic food, don't be surprised when your recommendations start sprouting quinoa recipes and farm-to-table restaurant reviews.
These algorithms aren't just guessing; they're learning and adapting in real time. Consider deep learning models, which use neural networks with many layers (hence the "deep" part) to process complex datasets and draw nuanced insights. These models excel at tasks like image and voice recognition but are equally adept at understanding user preferences and behaviours in a digital environment.
Incorporating machine learning algorithms into SEO strategies can revolutionise how content is personalised. By analysing search data, machine learning can predict trending keywords and topics, allowing marketers to create content that meets user interests before they even search for it. This proactive approach not only drives traffic but also enhances user engagement and conversion rates.
Machine learning's prowess isn't limited to content personalisation. It extends to pricing strategies as well. Dynamic pricing algorithms adjust prices based on demand, user behaviour, and competitive pricing, ensuring that each user gets the optimal price point to maximise conversions. It's like having a digital auctioneer, setting the perfect price for each bidder in real time.
Implementing these sophisticated algorithms requires a robust technical foundation. Collaboration with website services teams ensures that the infrastructure can support the high computational demands of real-time data processing and personalisation. Additionally, integrating these algorithms with a cost-per-lead estimator can provide deeper insights into the ROI of personalisation strategies, aligning marketing efforts with business goals.
It's essential to remember that personalisation, while powerful, must be handled with care. Data privacy concerns and regulations like GDPR necessitate transparent data practices and user consent. The challenge is balancing personalised experiences with respecting user privacy, a task that sophisticated machine learning algorithms can help manage by anonymising data and ensuring compliance.
To illustrate the impact, consider Netflix's recommendation engine, a paragon of machine learning in action. By leveraging algorithms like collaborative and content-based filtering, Netflix personalises its vast library for each user, significantly boosting engagement and retention. According to Netflix, its recommendation system is worth over $1 billion per year, underscoring the financial benefits of effective personalisation.
In conclusion, leveraging machine learning algorithms for personalisation transforms digital interactions from mundane to magical. These algorithms analyse user data to deliver tailored experiences that drive engagement, loyalty, and conversions. Whether it's predicting content preferences, adjusting prices dynamically, or enhancing SEO strategies, machine learning is the engine powering the next wave of digital personalisation. So, if you're ready to unlock the full potential of your website, dive into the world of machine learning algorithms – where personalisation is precise, proactive, and profoundly impactful.
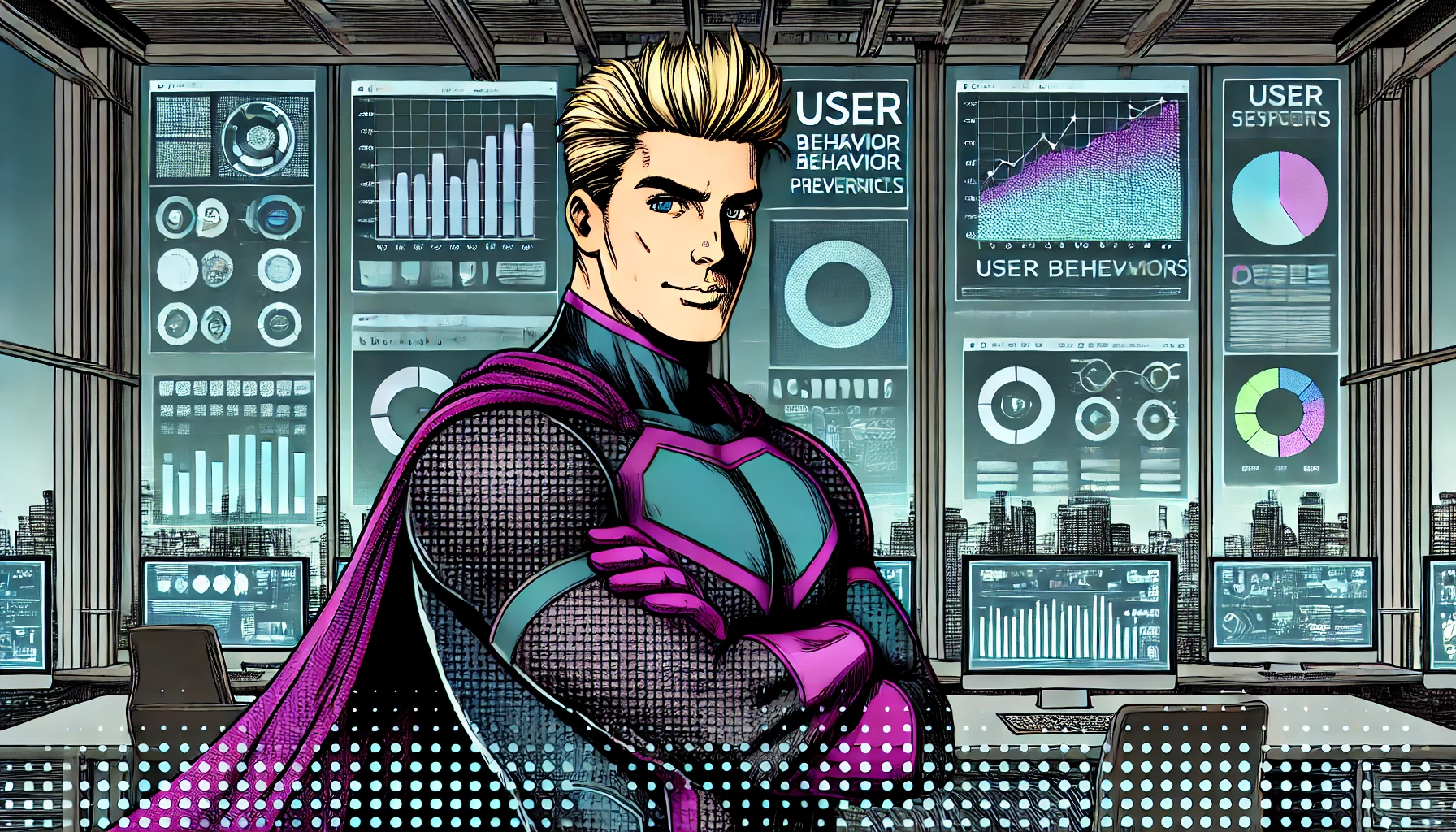
Implementing Behavioural Targeting to Customise User Experience
Imagine walking into your favourite coffee shop, and before you even order, the barista hands you your go-to cappuccino, perfectly crafted. That's behavioural targeting for you, but in the digital sphere. It’s all about creating a user experience so intuitive and personalised that it feels almost serendipitous.
Behavioural targeting utilises detailed analysis of users' online activities—clicks, page views, past purchases, and time spent on specific pages—to serve content and offers tailored specifically to them. This technique surpasses the conventional cookie-cutter approach, diving deep into user behaviour to deliver a personalised journey. The endgame? A boost in engagement, conversion rates, and overall user satisfaction.
At the core of behavioural targeting are data collection and segmentation. Using tools like cookies, pixels, and other tracking technologies, websites gather extensive data on user interactions. These interactions are then segmented into various behavioural cohorts. For instance, a user who frequently browses high-end gadgets but never makes a purchase might be served ads highlighting exclusive discounts or financing options, nudging them closer to a decision.
The efficacy of behavioural targeting is underscored by its dynamic nature. Unlike static targeting methods, which might categorise users based on a single demographic attribute, behavioural targeting adapts in real time. As user behaviours evolve, so do the targeting strategies, ensuring relevance and timeliness. It's like having a personal shopper who learns your preferences with every visit and adjusts recommendations accordingly.
Integrating behavioural targeting into Google Ads campaigns can significantly enhance ad performance. By focusing on user behaviours rather than generic demographics, ads become more relevant, leading to higher click-through rates and conversion rates. This approach also helps in optimizing ad spend, ensuring that budgets are allocated to audiences most likely to convert.
The role of machine learning in behavioural targeting cannot be overstated. Advanced algorithms analyse user data to identify patterns and predict future behaviours. For instance, an algorithm might detect that users who read certain types of blog posts are more likely to purchase a related product within a week. Armed with this insight, marketers can craft targeted content and offers to accelerate the purchase process.
Behavioural targeting isn't just about pushing products; it's about enhancing the overall user experience. A visitor to an e-commerce site might see personalised product recommendations based on their browsing history, while a content platform might suggest articles aligned with the user's reading habits. This personalised approach keeps users engaged, reducing bounce rates and increasing time spent on the site.
However, the implementation of behavioural targeting must be handled with finesse. Privacy concerns are paramount, and adhering to regulations like GDPR is non-negotiable. Users should be informed about data collection practices and given the option to opt-out. Transparency builds trust, and trust is the bedrock of any successful personalisation strategy.
For businesses looking to integrate behavioural targeting, a robust data management platform (DMP) is essential. A DMP aggregates and analyses user data from various sources, enabling precise targeting. Collaboration with SEO services ensures that the website infrastructure can support these advanced targeting techniques, maintaining performance and user experience.
Case in point: Amazon. The retail giant's success is significantly attributed to its sophisticated behavioural targeting mechanisms. By analysing user behaviour, Amazon serves personalised product recommendations, tailored ads, and even customised email marketing campaigns. This relentless focus on personalisation drives engagement, loyalty, and repeat purchases.
Implementing behavioural targeting can also benefit small business SEO efforts. By understanding user behaviour, small businesses can create more relevant content, optimise keyword strategies, and enhance user experience, levelling the playing field against larger competitors.
Moreover, behavioural targeting can be effectively utilised in social media advertising. Platforms like Facebook and Instagram offer advanced targeting options based on user behaviour, enabling marketers to reach highly specific audiences with personalised ads. This precision targeting enhances ad relevance, engagement, and conversion rates.
To sum up, behavioural targeting transforms digital marketing from a broad-spectrum approach to a precision-guided strategy. By leveraging detailed user data, businesses can deliver personalised experiences that resonate with individual users, driving engagement and conversions. As technology evolves, those who master behavioural targeting will find themselves at the forefront of digital marketing innovation, creating user experiences that are not just personalised but profoundly impactful.
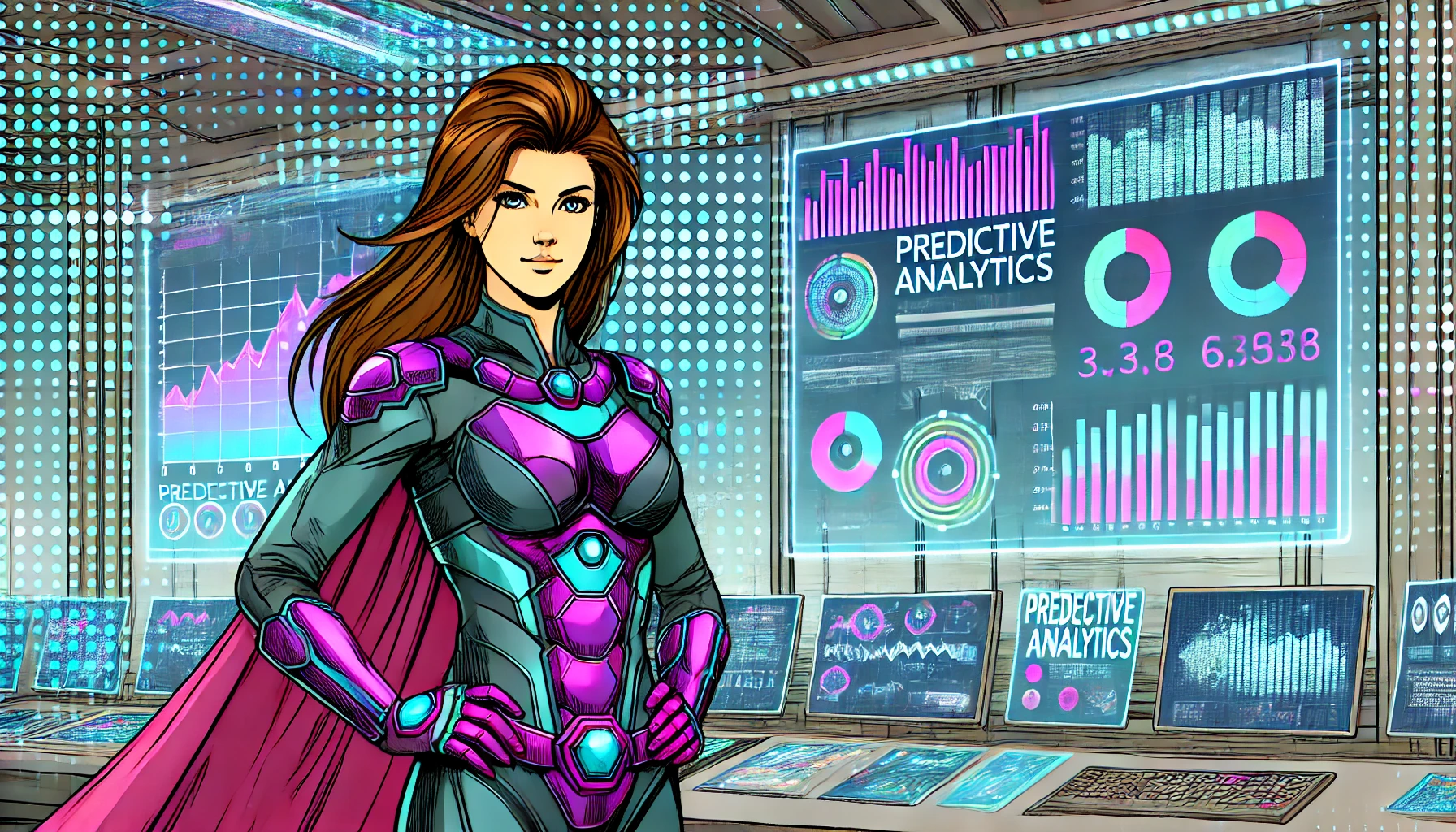
Using Predictive Analytics for Tailored Content Delivery
Imagine your website as a savvy butler, anticipating every need of your visitors before they even utter a word. That's the magic of predictive analytics in tailored content delivery—where every interaction feels as though the website has read your mind, offering exactly what you want when you want it.
Predictive analytics leverages historical data, statistical algorithms, and machine learning techniques to identify future outcomes based on past behaviours. In the context of content delivery, it means analysing user data to predict what content will most likely engage each visitor. The result is a highly personalised user experience that increases engagement, satisfaction, and conversion rates.
The first step in harnessing predictive analytics for content delivery is data collection. This involves gathering extensive data on user interactions, such as page views, click patterns, time spent on content, and previous purchases. Advanced analytics platforms track this data across multiple touchpoints, building a comprehensive profile for each user. This data feeds into machine learning models that identify patterns and trends, enabling precise predictions about what content will resonate with each visitor.
Predictive modelling techniques, such as regression analysis, decision trees, and neural networks, play a crucial role in this process. For instance, regression analysis might reveal that users who engage with certain types of articles are more likely to be interested in related product recommendations. Decision trees can segment users into different cohorts based on their behaviours, while neural networks can identify more complex patterns and relationships in the data.
Integrating predictive analytics with your website services ensures that these models run efficiently and effectively, delivering real-time content recommendations. This requires robust infrastructure capable of processing large datasets and generating predictions on the fly. Collaboration with technical SEO experts can optimise your site’s performance, ensuring that personalised content is delivered seamlessly.
A practical application of predictive analytics in content delivery is personalised homepage experiences. By analysing a user's past interactions, the predictive model can determine which sections of the homepage are most relevant. For a returning visitor interested in fitness, the homepage might prioritise workout articles and healthy recipes, while for a tech enthusiast, it might showcase the latest gadget reviews. This dynamic adaptation not only enhances user engagement but also reduces bounce rates.
Predictive analytics also excels in email marketing. By analysing past email interactions, such as opens and clicks, predictive models can determine the optimal content, timing, and frequency for each user. This means that instead of sending generic newsletters, you can deliver highly targeted emails that align with the user’s interests and behaviours, significantly boosting open and click-through rates.
Another powerful application is in e-commerce SEO. Predictive analytics can identify trends in search data, helping to forecast popular products and keywords. This foresight enables e-commerce sites to optimise product listings and content for emerging trends, capturing organic traffic and driving conversions. Additionally, predictive models can tailor product recommendations based on a user’s browsing and purchase history, creating a shopping experience that feels uniquely curated.
Social media platforms also benefit from predictive analytics. By analysing user interactions, predictive models can determine which types of posts are most likely to engage each user. This insight allows for the creation of personalised social media feeds that keep users engaged longer. Platforms like Facebook and Instagram already leverage these techniques to personalise the content users see, enhancing user satisfaction and platform loyalty.
Moreover, predictive analytics can significantly enhance the effectiveness of advertising campaigns. By predicting which users are most likely to convert, marketers can target ads more precisely, optimising ad spend and increasing ROI. This approach also helps in creating personalised ad content that resonates with the target audience, driving higher engagement and conversion rates.
However, implementing predictive analytics for tailored content delivery requires careful consideration of data privacy. Compliance with regulations such as GDPR is essential to ensure that user data is collected and used responsibly. Transparency in data practices builds user trust, which is crucial for the long-term success of personalisation strategies.
The benefits of predictive analytics in content delivery are well-documented. According to a report by Salesforce, high-performing marketers are 7.5 times more likely to leverage predictive intelligence to enhance their content marketing efforts. Additionally, McKinsey reports that personalisation can reduce acquisition costs by as much as 50%, lift revenues by 5 to 15%, and increase the efficiency of marketing spend by 10 to 30%.
In conclusion, using predictive analytics for tailored content delivery transforms the user experience from generic to exceptional. By leveraging advanced data analysis and machine learning models, businesses can predict user preferences and deliver content that engages and converts. As technology continues to evolve, those who embrace predictive analytics will lead the way in creating highly personalised, impactful user experiences.
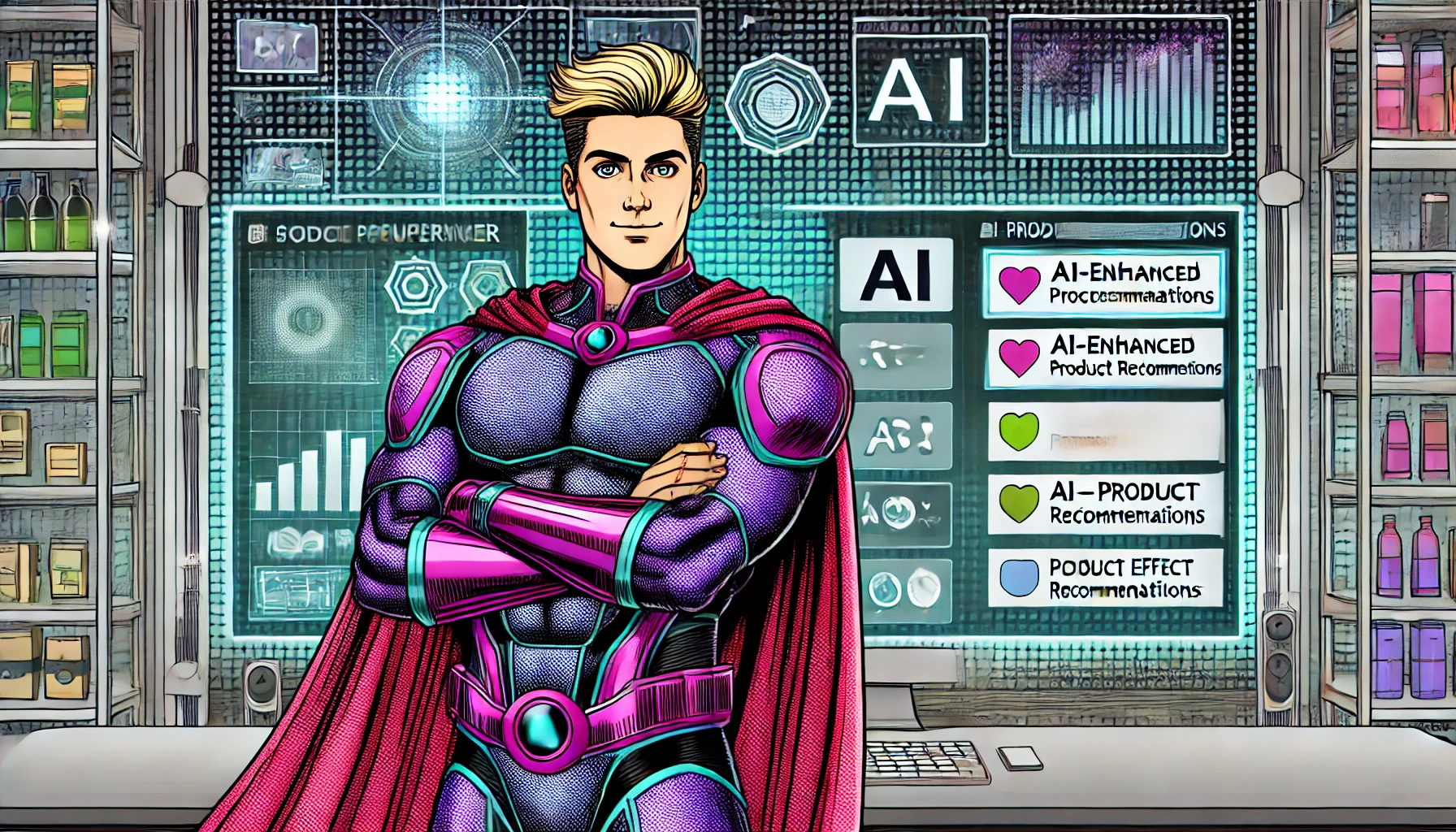
Enhancing Product Recommendations with AI
Imagine walking into a store where the shopkeeper knows your preferences so well that every suggestion feels like it was made just for you. That’s the level of personalisation AI brings to product recommendations—transforming the online shopping experience from a generic aisle wander into a curated showcase of exactly what you didn’t know you needed.
AI-driven product recommendations are the digital equivalent of a personal shopper with an impeccable memory and unerring instincts. These systems leverage advanced algorithms and vast amounts of data to predict what products will appeal to each user, boosting engagement, satisfaction, and ultimately, sales. The magic lies in the blend of collaborative filtering, content-based filtering, and deep learning models that continually refine and enhance these recommendations.
At the heart of AI-powered recommendations is collaborative filtering, a technique that identifies patterns in user behaviour by analysing the interactions of many users. For instance, if User A and User B have similar browsing and purchasing habits, and User A buys a new item, User B will likely receive a recommendation for that item. This "wisdom of the crowd" approach underpins the success of recommendation engines on platforms like Amazon and Netflix.
Content-based filtering takes a more individual approach, analysing the attributes of items a user has interacted with to suggest similar items. This technique might consider the categories, tags, and keywords associated with products a user has viewed or purchased, creating a profile of their preferences. For example, a user who frequently buys eco-friendly products will receive recommendations for other sustainable items.
Deep learning, with its neural networks and layers of abstraction, further enhances these systems. These models can process vast amounts of data to understand complex patterns and preferences that simpler algorithms might miss. By considering not just direct interactions but also indirect signals—like the time spent on product pages or the sequence of pages visited—deep learning models can make uncannily accurate predictions about what users will find appealing.
Integrating these AI technologies into your e-commerce SEO strategy can dramatically improve product visibility and relevance. By analysing search trends and user behaviour, AI can optimise product listings and meta tags, ensuring that the most relevant products are recommended to the right users at the right time. This proactive approach not only drives traffic but also increases conversion rates by aligning product recommendations with user intent.
Moreover, AI-powered recommendations are not confined to the digital domain. They can seamlessly integrate with Google Ads campaigns, ensuring that the ads users see are precisely targeted based on their browsing history and purchase patterns. This level of personalisation increases the likelihood of click-throughs and conversions, maximising the return on ad spend.
The application of AI in product recommendations extends beyond merely suggesting products. It encompasses dynamic pricing strategies, where machine learning algorithms adjust prices based on demand, user behaviour, and competitive pricing. This real-time adaptability ensures that prices are optimised to maximise sales and profit margins, much like having a digital market analyst working around the clock.
For businesses looking to enhance their recommendation engines, collaboration with technical SEO experts is crucial. These experts ensure that the website’s infrastructure can handle the computational demands of AI algorithms and that data pipelines are robust and efficient. The synergy between technical SEO and AI-driven recommendations results in a seamless, responsive user experience.
Real-world examples highlight the transformative impact of AI on product recommendations. Take Spotify, which uses AI to personalise music recommendations through its Discover Weekly and Daily Mix playlists. By analysing listening habits, skip rates, and user engagement, Spotify's algorithms curate playlists that feel tailor-made, keeping users engaged and loyal. This approach has significantly contributed to Spotify's growth and user retention.
Similarly, Netflix's recommendation engine is a masterclass in AI application. By leveraging collaborative filtering and deep learning, Netflix personalises content recommendations, keeping users hooked and reducing churn. According to Netflix, its recommendation system saves the company over $1 billion annually by reducing cancellations and driving engagement.
However, implementing AI-driven recommendations requires careful consideration of data privacy. Adhering to regulations such as GDPR is paramount to ensure that user data is collected and used responsibly. Transparent data practices and user consent are crucial for building trust, which is the foundation of successful personalisation strategies.
In the future, the evolution of AI will further refine product recommendations. Emerging technologies like reinforcement learning, which adapts based on continuous feedback, will create even more personalised and contextually relevant recommendations. This evolution will enhance user satisfaction and loyalty, setting new standards in the e-commerce landscape.
In conclusion, enhancing product recommendations with AI transforms the shopping experience from mundane to magical. By leveraging collaborative filtering, content-based filtering, and deep learning, businesses can deliver highly personalised recommendations that resonate with users. Integrating these technologies with e-commerce SEO and Google Ads campaigns ensures that product recommendations are not only relevant but also strategically aligned with user intent. As AI continues to evolve, those who harness its potential will lead the charge in creating exceptional, personalised user experiences that drive engagement and sales.
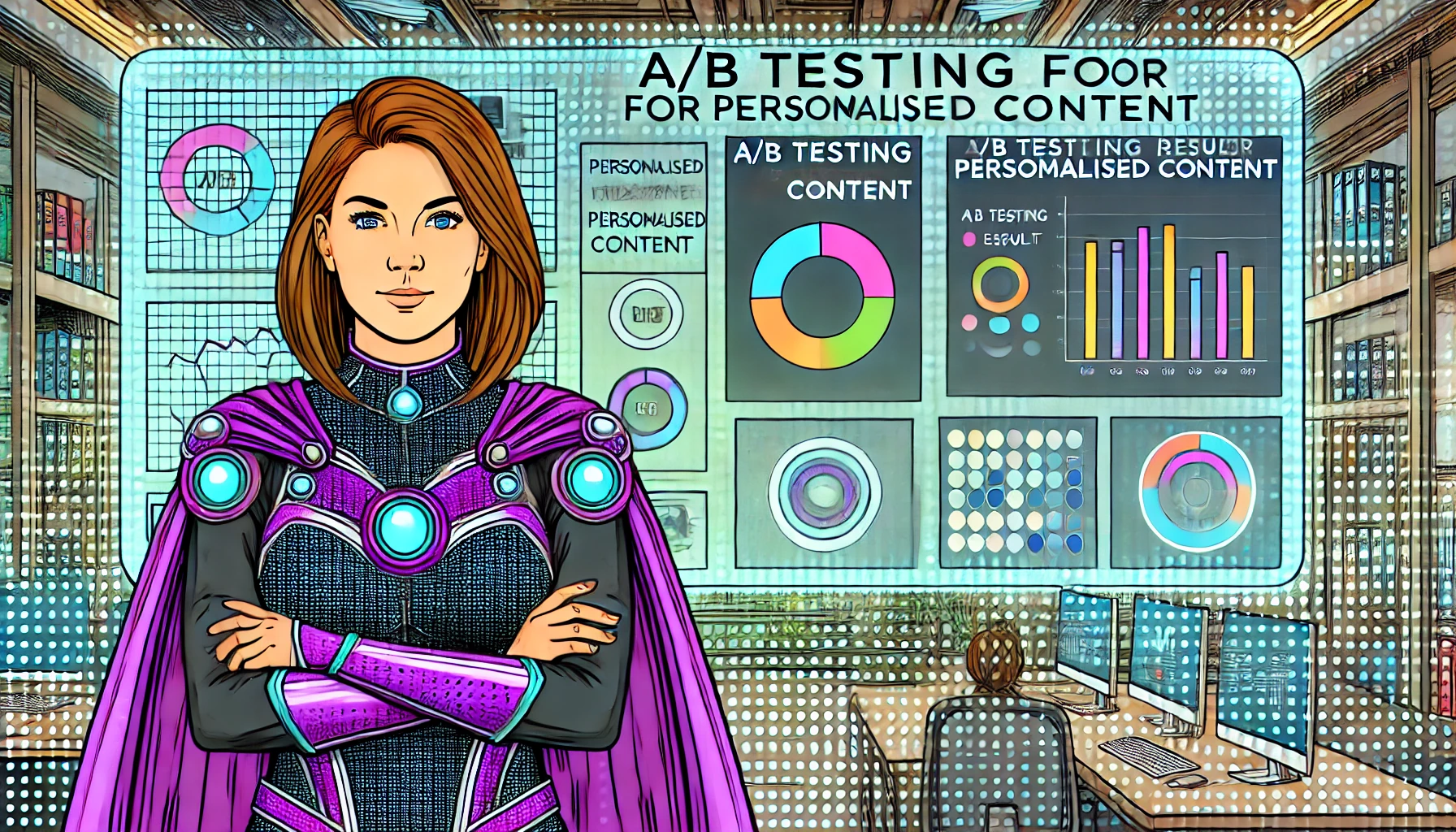
A/B Testing Personalised Content for Conversion Optimisation
A/B testing personalised content is akin to running a scientific experiment, except the goal is to make your website more charming and persuasive, rather than discovering new elements or solving equations. It’s about determining which personalised experience most effectively nudges users toward conversion, whether that’s making a purchase, signing up for a newsletter, or engaging with content.
A/B testing, also known as split testing, involves comparing two versions of a webpage or content piece to see which performs better. By segmenting your audience and showing different versions to different groups, you can measure the impact of specific changes on user behaviour. When applied to personalised content, A/B testing becomes a powerful tool to refine and optimise the user experience.
To get started, identify the key metrics you want to improve. These could include click-through rates, conversion rates, bounce rates, or time on page. Next, decide what elements of your personalised content you want to test. This could range from headlines, images, and calls-to-action to more sophisticated elements like product recommendations or dynamic content sections.
One effective approach is to test different levels of personalisation. For instance, compare a control group experiencing a generic version of your site against a test group exposed to highly personalised content. This could involve tailored product recommendations based on browsing history, personalised greetings, or dynamic content blocks that adjust based on user preferences.
Integrating machine learning algorithms enhances the personalisation and A/B testing process. These algorithms can help identify which user segments are most responsive to different types of content, enabling more precise targeting and segmentation for your tests. By leveraging predictive analytics, you can anticipate which personalisation strategies are likely to be most effective, refining your tests for maximum impact.
Consider an example: an e-commerce site testing personalised product recommendations. The control group sees generic recommendations, while the test group receives recommendations based on their past browsing and purchase history. By tracking conversion rates, average order values, and user engagement, you can determine which approach drives better results. This process can be further refined by testing different recommendation algorithms, such as collaborative filtering versus content-based filtering.
Another scenario could involve testing personalised email campaigns. Segment your audience and send different versions of an email based on user behaviour data. One version could feature personalised product suggestions, while another might highlight personalised content based on previous interactions. By measuring open rates, click-through rates, and conversion rates, you can identify the most effective personalisation strategy.
A/B testing personalised content isn’t just about what works better; it’s also about understanding why. Detailed analytics and user feedback can provide insights into user preferences and behaviours, helping to inform future personalisation strategies. Tools like heatmaps and session recordings can reveal how users interact with different versions of your content, providing qualitative data to complement your quantitative results.
It’s essential to run A/B tests for a sufficient duration to gather meaningful data. The length of your test will depend on your traffic volume and the statistical significance of your results. Using tools like Optimizely or Google Optimize can streamline the process, providing robust platforms for setting up, running, and analysing your A/B tests.
While A/B testing personalised content, it's crucial to ensure your site infrastructure can support dynamic content delivery and real-time data processing. Collaborating with technical SEO experts ensures your site remains performant, even as you introduce complex personalisation and testing mechanisms.
Privacy and data protection should also be top priorities. Compliance with regulations like GDPR is essential, particularly when dealing with personalised content that relies on user data. Ensuring transparency in data collection and usage builds trust with your users, which is fundamental for successful personalisation.
One real-world example of effective A/B testing for personalisation is Netflix. By continuously testing different personalised content recommendations, Netflix optimises its user interface and content delivery, enhancing user engagement and retention. Each test provides insights that help Netflix refine its algorithms and user experience, contributing to its success as a leading streaming platform.
In conclusion, A/B testing personalised content is a strategic approach to conversion optimisation. By systematically comparing different versions of personalised content, businesses can identify the most effective strategies for engaging users and driving conversions. Integrating machine learning and predictive analytics further enhances the precision and impact of these tests. As personalisation becomes increasingly central to user experience, those who master A/B testing will be well-positioned to deliver superior, tailored experiences that resonate with their audience and drive business growth.

Integrating Personalisation with Email Marketing Campaigns
Integrating personalisation with email marketing campaigns is like adding a dash of magic to your outreach efforts—suddenly, your messages feel less like a marketing push and more like a thoughtful conversation. The result? Higher engagement, better open rates, and, crucially, more conversions. But how do you get from generic email blasts to highly personalised communications that make recipients feel genuinely seen and valued?
To start with, successful personalisation hinges on robust data collection and analysis. Gathering detailed information about your subscribers—such as their browsing history, purchase behaviour, and interaction with past emails—provides the foundation for personalised email campaigns. This data is the fuel that powers sophisticated segmentation and targeting strategies, transforming raw information into actionable insights.
Segmentation is the first step in creating personalised email campaigns. By dividing your email list into distinct groups based on specific criteria (such as demographics, purchase history, or engagement level), you can tailor your content to address the unique needs and interests of each segment. This ensures that your messages are relevant and timely, which is key to capturing the attention of your audience.
Next, leverage predictive analytics to anticipate what content will resonate most with each segment. Predictive models can analyse past behaviours to forecast future actions, enabling you to send highly relevant recommendations, offers, or content. For example, if a segment of your audience frequently buys eco-friendly products, you can predict that they might be interested in new sustainable offerings or related content.
Dynamic content is a game-changer in email personalisation. By incorporating elements that change based on user data, you can create emails that feel individually crafted for each recipient. This could include personalised product recommendations, location-specific offers, or content that aligns with the recipient's past interactions. Dynamic content ensures that each email is not only personalised but also contextually relevant.
For instance, integrating dynamic content into a Google Ads campaign ensures that the ads your subscribers see align with the content of your personalised emails. This cohesive approach reinforces your message across multiple touchpoints, enhancing the overall effectiveness of your marketing efforts.
Personalisation extends beyond just inserting the recipient’s name in the subject line. It's about creating a narrative that speaks directly to their interests and needs. Use machine learning algorithms to analyse user data and craft personalised subject lines, email copy, and calls to action. These algorithms can test different variations to determine which elements drive the highest engagement, continuously refining your approach based on real-time data.
Automation platforms like HubSpot, Mailchimp, and Salesforce Marketing Cloud are invaluable tools for implementing personalised email marketing campaigns. These platforms offer advanced segmentation, dynamic content capabilities, and integration with other marketing tools, allowing for a seamless, data-driven approach to email marketing.
One practical application is in e-commerce SEO. Personalised emails can significantly enhance the customer journey, from initial engagement to post-purchase follow-up. For instance, sending personalised product recommendations based on past purchases or browsing history can drive repeat purchases and increase customer loyalty. Additionally, abandoned cart emails with personalised content can recover potentially lost sales by reminding customers of the items they left behind, often with a tailored incentive to complete the purchase.
Another powerful strategy is to integrate personalisation with technical SEO to ensure that your website and email campaigns are aligned. For instance, personalised email content can be mirrored on personalised landing pages, creating a seamless and engaging experience from inbox to website. This ensures that the message consistency is maintained, enhancing user experience and boosting conversion rates.
Personalised email campaigns also benefit significantly from A/B testing. By testing different versions of personalised content, you can determine what resonates most with your audience. For example, you could test different subject lines, email layouts, or call-to-action buttons to see which variations drive higher open rates and conversions. These insights allow you to continually refine your approach, ensuring that your emails are always optimised for engagement.
Privacy and data security are paramount when implementing personalised email campaigns. Compliance with regulations like GDPR is essential to maintain user trust and avoid legal pitfalls. Ensuring transparency in how you collect and use data, and providing users with control over their preferences, helps build a positive relationship with your audience.
Real-world examples highlight the impact of personalised email campaigns. Amazon, for instance, excels in sending personalised product recommendations based on user behaviour. These emails drive significant revenue by showcasing items that users are likely to be interested in, based on their browsing and purchase history. Similarly, Spotify's personalised email campaigns, which include custom playlists and music recommendations, enhance user engagement and retention.
In conclusion, integrating personalisation with email marketing campaigns transforms generic outreach into a powerful, user-centric strategy. By leveraging data, predictive analytics, dynamic content, and advanced automation tools, businesses can create highly personalised email experiences that resonate with recipients, drive engagement, and boost conversions. As technology evolves, those who master personalised email marketing will find themselves at the forefront of digital engagement, delivering messages that not only capture attention but also build lasting relationships with their audience.
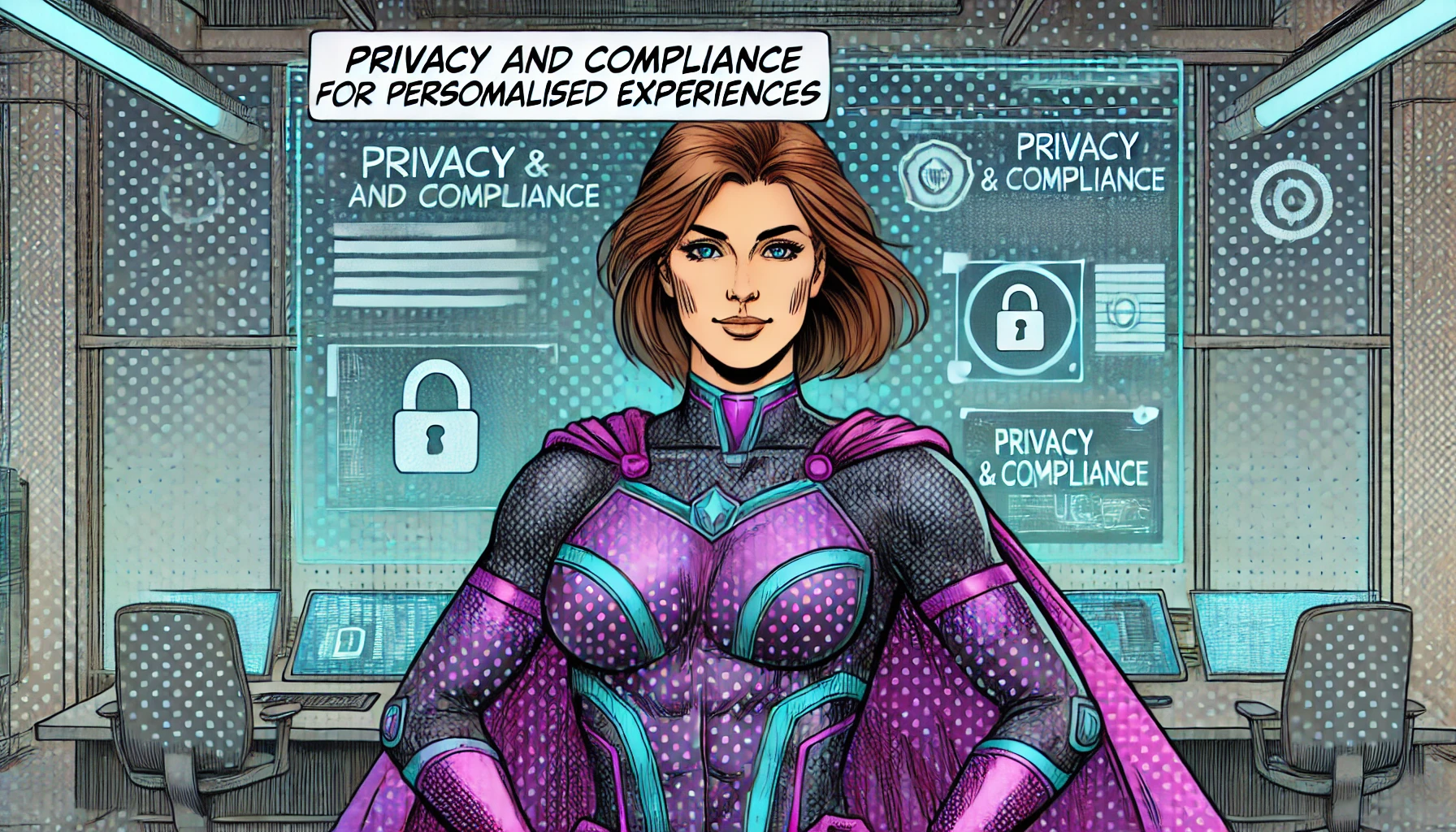
Ensuring Privacy and Compliance in Personalised Experiences
Crafting personalised experiences is like being a tailor who not only knows your exact measurements but also respects your privacy preferences. Balancing personalisation with privacy is essential to building trust and maintaining compliance with ever-evolving data protection regulations. Let's explore how to deliver those bespoke digital experiences while ensuring privacy and compliance.
First, understand that privacy and personalisation can coexist. They are not mutually exclusive but require a framework that respects user data and complies with legal standards. The cornerstone of this framework is transparency. Users need to know what data is being collected, how it's being used, and for what purposes. This means clear, concise privacy policies and easy-to-understand consent forms.
Consent is the bedrock of privacy compliance. Under regulations like GDPR, explicit consent is required before collecting or using personal data for personalisation. This involves clear opt-in mechanisms where users actively agree to data collection, rather than pre-checked boxes or passive acceptance. Additionally, providing users with the option to withdraw consent at any time is crucial. This ensures that personalisation efforts are both compliant and respectful of user autonomy.
Data minimisation is another critical principle. Collect only the data necessary to achieve personalisation goals. This not only reduces the risk of data breaches but also aligns with regulations that mandate minimal data collection. For instance, if you are personalising email campaigns, focus on collecting email interactions and preferences rather than unnecessary personal details.
Anonymisation and pseudonymisation are effective strategies to enhance privacy. By anonymising data, personal identifiers are removed, making it difficult to trace data back to an individual. Pseudonymisation replaces identifiable information with pseudonyms, ensuring that data can be used for analysis and personalisation without compromising privacy. These techniques are especially valuable in sectors where data sensitivity is paramount.
Secure data storage and transmission are non-negotiable. Implement robust encryption protocols for data at rest and in transit to prevent unauthorised access. Regular security audits and compliance checks should be conducted to identify and address vulnerabilities. Collaborating with technical SEO experts ensures that your website's infrastructure is secure, supporting safe data handling practices.
Transparency extends beyond consent forms and privacy policies. It involves ongoing communication with users about how their data is used and the benefits of data-driven personalisation. Regular updates and educational content about privacy practices can build trust and foster a positive relationship with your audience.
A practical application of these principles is seen in Google Ads campaigns. By ensuring that user data collected for ad personalisation is obtained with explicit consent, anonymised, and securely stored, businesses can comply with privacy regulations while delivering relevant ads. This approach not only enhances compliance but also improves ad performance by building user trust.
Another critical aspect of ensuring privacy and compliance is the implementation of robust data governance frameworks. This involves establishing clear policies and procedures for data handling, access controls, and regular audits. A data governance framework ensures that all personalisation efforts are aligned with regulatory requirements and best practices.
For instance, when integrating personalisation into e-commerce SEO, ensuring that user data collected for personalisation is handled according to strict data governance policies is essential. This might include setting access controls to restrict data access to authorised personnel only and conducting regular compliance checks to ensure adherence to data protection regulations.
User-centric design is another key component. Implement privacy by design and by default, ensuring that privacy considerations are embedded into the development of personalisation strategies from the outset. This approach not only helps in meeting compliance requirements but also enhances user experience by making privacy a fundamental feature rather than an afterthought.
Real-world examples illustrate the importance of privacy and compliance in personalisation. Apple’s introduction of App Tracking Transparency (ATT) is a notable case. By requiring apps to obtain explicit permission before tracking user activity, Apple has set a new standard for privacy in personalisation. This move not only ensures compliance with global privacy regulations but also aligns with user expectations for transparency and control over their data.
Similarly, GDPR compliance has driven significant changes in how businesses approach personalisation. Companies like Spotify and Netflix have implemented robust consent mechanisms and transparent data practices to comply with GDPR while continuing to deliver highly personalised user experiences. These efforts demonstrate that it is possible to achieve personalisation without compromising on privacy.
In conclusion, ensuring privacy and compliance in personalised experiences requires a multi-faceted approach. It involves obtaining explicit consent, practising data minimisation, employing anonymisation and pseudonymisation, securing data storage and transmission, and implementing robust data governance frameworks. By embedding privacy considerations into the design and execution of personalisation strategies, businesses can build trust, comply with regulations, and deliver exceptional user experiences. As privacy regulations continue to evolve, staying ahead of compliance requirements while maintaining a focus on user-centric personalisation will be key to long-term success.
External References:
- GDPR Compliance
- Apple’s App Tracking Transparency
- Google Ads Data Protection
- Anonymisation Techniques
- Pseudonymisation Explained
- Data Minimisation Principle
- Privacy by Design
- Spotify GDPR Compliance
- HubSpot Email Marketing
- Mailchimp Personalisation Features
- Salesforce Marketing Cloud
- Amazon Personalisation Case Study
- Spotify Personalised Email Campaigns
- Dynamic Content in Email Marketing
- GDPR Compliance
- Predictive Analytics in Email Marketing